了解了模型大致原理,我們可以詳細的看一下究竟Self-Attention結(jié)構(gòu)是怎樣的。其基本結(jié)構(gòu)如下
對于self-attention來講,Q(Query), K(Key), V(Value)三個矩陣均來自同一輸入,首先我們要計算Q與K之間的點乘,然后為了防止其結(jié)果過大,會除以一個尺度標(biāo)度,其中為一個query和key向量的維度。再利用Softmax操作將其結(jié)果歸一化為概率分布,然后再乘以矩陣V就得到權(quán)重求和的表示。該操作可以表示為
這里可能比較抽象,我們來看一個具體的例子(圖片來源于https://jalammar.github.io/illustrated-transformer/),該博客講解的極其清晰,強烈推薦),假如我們要翻譯一個詞組Thinking Machines,其中Thinking的輸入的embedding vector用表示,Machines的embedding vector用表示。
當(dāng)我們處理Thinking這個詞時,我們需要計算句子中所有詞與它的Attention Score,這就像將當(dāng)前詞作為搜索的query,去和句子中所有詞(包含該詞本身)的key去匹配,看看相關(guān)度有多高。我們用代表Thinking對應(yīng)的query vector,及分別代表Thinking以及Machines對應(yīng)的key vector,則計算Thinking的attention score的時候我們需要計算與的點乘,同理,我們計算Machines的attention score的時候需要計算與的點乘。如上圖中所示我們分別得到了與的點乘積,然后我們進行尺度縮放與softmax歸一化,如下圖所示:
顯然,當(dāng)前單詞與其自身的attention score一般最大,其他單詞根據(jù)與當(dāng)前單詞重要程度有相應(yīng)的score。然后我們在用這些attention score與value vector相乘,得到加權(quán)的向量。
如果將輸入的所有向量合并為矩陣形式,則所有query, key, value向量也可以合并為矩陣形式表示:
其中是我們模型訓(xùn)練過程學(xué)習(xí)到的合適的參數(shù)。上述操作即可簡化為矩陣形式:
二、Self_Attention模型搭建
筆者使用Keras來實現(xiàn)對于Self_Attention模型的搭建,由于網(wǎng)絡(luò)中間參數(shù)量比較多,這里采用自定義網(wǎng)絡(luò)層的方法構(gòu)建Self_Attention。
Keras實現(xiàn)自定義網(wǎng)絡(luò)層。需要實現(xiàn)以下三個方法:(注意input_shape是包含batch_size項的)
build(input_shape): 這是你定義權(quán)重的地方。這個方法必須設(shè)self.built = True,可以通過調(diào)用super([Layer], self).build()完成。
call(x): 這里是編寫層的功能邏輯的地方。你只需要關(guān)注傳入call的第一個參數(shù):輸入張量,除非你希望你的層支持masking。
compute_output_shape(input_shape): 如果你的層更改了輸入張量的形狀,你應(yīng)該在這里定義形狀變化的邏輯,這讓Keras能夠自動推斷各層的形狀。
實現(xiàn)代碼如下:
from keras.preprocessing import sequencefrom keras.datasets import imdbfrom matplotlib import pyplot as pltimport pandas as pdfrom keras import backend as Kfrom keras.engine.topology import Layerclass Self_Attention(Layer): def __init__(self, output_dim, **kwargs): self.output_dim = output_dim super(Self_Attention, self).__init__(**kwargs) def build(self, input_shape): # 為該層創(chuàng)建一個可訓(xùn)練的權(quán)重 #inputs.shape = (batch_size, time_steps, seq_len) self.kernel = self.add_weight(name='kernel', shape=(3,input_shape[2], self.output_dim), initializer='uniform', trainable=True) super(Self_Attention, self).build(input_shape) # 一定要在最后調(diào)用它 def call(self, x): WQ = K.dot(x, self.kernel[0]) WK = K.dot(x, self.kernel[1]) WV = K.dot(x, self.kernel[2]) print("WQ.shape",WQ.shape) print("K.permute_dimensions(WK, [0, 2, 1]).shape",K.permute_dimensions(WK, [0, 2, 1]).shape) QK = K.batch_dot(WQ,K.permute_dimensions(WK, [0, 2, 1])) QK = QK / (64**0.5) QK = K.softmax(QK) print("QK.shape",QK.shape) V = K.batch_dot(QK,WV) return V def compute_output_shape(self, input_shape): return (input_shape[0],input_shape[1],self.output_dim)
這里可以對照一中的概念講解來理解代碼
如果將輸入的所有向量合并為矩陣形式,則所有query, key, value向量也可以合并為矩陣形式表示
上述內(nèi)容對應(yīng)
WQ = K.dot(x, self.kernel[0])WK = K.dot(x, self.kernel[1])WV = K.dot(x, self.kernel[2])
其中是我們模型訓(xùn)練過程學(xué)習(xí)到的合適的參數(shù)。上述操作即可簡化為矩陣形式:
上述內(nèi)容對應(yīng)(為什么使用batch_dot呢?這是由于input_shape是包含batch_size項的)
QK = K.batch_dot(WQ,K.permute_dimensions(WK, [0, 2, 1]))QK = QK / (64**0.5)QK = K.softmax(QK)print("QK.shape",QK.shape)V = K.batch_dot(QK,WV)
這里QK = QK / (64**0.5) 是除以一個歸一化系數(shù),(64**0.5)是筆者自己定義的,其他文章可能會采用不同的方法。
三、訓(xùn)練網(wǎng)絡(luò)
項目完整代碼如下,這里使用的是Keras自帶的imdb影評數(shù)據(jù)集。
#%%from keras.preprocessing import sequencefrom keras.datasets import imdbfrom matplotlib import pyplot as pltimport pandas as pdfrom keras import backend as Kfrom keras.engine.topology import Layerclass Self_Attention(Layer): def __init__(self, output_dim, **kwargs): self.output_dim = output_dim super(Self_Attention, self).__init__(**kwargs) def build(self, input_shape): # 為該層創(chuàng)建一個可訓(xùn)練的權(quán)重 #inputs.shape = (batch_size, time_steps, seq_len) self.kernel = self.add_weight(name='kernel', shape=(3,input_shape[2], self.output_dim), initializer='uniform', trainable=True) super(Self_Attention, self).build(input_shape) # 一定要在最后調(diào)用它 def call(self, x): WQ = K.dot(x, self.kernel[0]) WK = K.dot(x, self.kernel[1]) WV = K.dot(x, self.kernel[2]) print("WQ.shape",WQ.shape) print("K.permute_dimensions(WK, [0, 2, 1]).shape",K.permute_dimensions(WK, [0, 2, 1]).shape) QK = K.batch_dot(WQ,K.permute_dimensions(WK, [0, 2, 1])) QK = QK / (64**0.5) QK = K.softmax(QK) print("QK.shape",QK.shape) V = K.batch_dot(QK,WV) return V def compute_output_shape(self, input_shape): return (input_shape[0],input_shape[1],self.output_dim)max_features = 20000print('Loading data...')(x_train, y_train), (x_test, y_test) = imdb.load_data(num_words=max_features)#標(biāo)簽轉(zhuǎn)換為獨熱碼y_train, y_test = pd.get_dummies(y_train),pd.get_dummies(y_test)print(len(x_train), 'train sequences')print(len(x_test), 'test sequences')#%%數(shù)據(jù)歸一化處理maxlen = 64print('Pad sequences (samples x time)')x_train = sequence.pad_sequences(x_train, maxlen=maxlen)x_test = sequence.pad_sequences(x_test, maxlen=maxlen)print('x_train shape:', x_train.shape)print('x_test shape:', x_test.shape)#%%batch_size = 32from keras.models import Modelfrom keras.optimizers import SGD,Adamfrom keras.layers import *from Attention_keras import Attention,Position_EmbeddingS_inputs = Input(shape=(64,), dtype='int32')embeddings = Embedding(max_features, 128)(S_inputs)O_seq = Self_Attention(128)(embeddings)O_seq = GlobalAveragePooling1D()(O_seq)O_seq = Dropout(0.5)(O_seq)outputs = Dense(2, activation='softmax')(O_seq)model = Model(inputs=S_inputs, outputs=outputs)print(model.summary())# try using different optimizers and different optimizer configsopt = Adam(lr=0.0002,decay=0.00001)loss = 'categorical_crossentropy'model.compile(loss=loss, optimizer=opt, metrics=['accuracy'])#%%print('Train...')h = model.fit(x_train, y_train, batch_size=batch_size, epochs=5, validation_data=(x_test, y_test))plt.plot(h.history["loss"],label="train_loss")plt.plot(h.history["val_loss"],label="val_loss")plt.plot(h.history["acc"],label="train_acc")plt.plot(h.history["val_acc"],label="val_acc")plt.legend()plt.show()#model.save("imdb.h5")
四、結(jié)果輸出
(TF_GPU) D:FilesDATAsprjspython f_keras ransfromerdemo>C:/Files/APPs/RuanJian/Miniconda3/envs/TF_GPU/python.exe d:/Files/DATAs/prjs/python/tf_keras/transfromerdemo/train.1.pyUsing TensorFlow backend.Loading data...25000 train sequences25000 test sequencesPad sequences (samples x time)x_train shape: (25000, 64)x_test shape: (25000, 64)WQ.shape (?, 64, 128)K.permute_dimensions(WK, [0, 2, 1]).shape (?, 128, 64)QK.shape (?, 64, 64)_________________________________________________________________Layer (type) Output Shape Param #=================================================================input_1 (InputLayer) (None, 64) 0_________________________________________________________________embedding_1 (Embedding) (None, 64, 128) 2560000_________________________________________________________________self__attention_1 (Self_Atte (None, 64, 128) 49152_________________________________________________________________global_average_pooling1d_1 ( (None, 128) 0_________________________________________________________________dropout_1 (Dropout) (None, 128) 0_________________________________________________________________dense_1 (Dense) (None, 2) 258=================================================================Total params: 2,609,410Trainable params: 2,609,410Non-trainable params: 0_________________________________________________________________NoneTrain...Train on 25000 samples, validate on 25000 samplesEpoch 1/525000/25000 [==============================] - 17s 693us/step - loss: 0.5244 - acc: 0.7514 - val_loss: 0.3834 - val_acc: 0.8278Epoch 2/525000/25000 [==============================] - 15s 615us/step - loss: 0.3257 - acc: 0.8593 - val_loss: 0.3689 - val_acc: 0.8368Epoch 3/525000/25000 [==============================] - 15s 614us/step - loss: 0.2602 - acc: 0.8942 - val_loss: 0.3909 - val_acc: 0.8303Epoch 4/525000/25000 [==============================] - 15s 618us/step - loss: 0.2078 - acc: 0.9179 - val_loss: 0.4482 - val_acc: 0.8215Epoch 5/525000/25000 [==============================] - 15s 619us/step - loss: 0.1639 - acc: 0.9368 - val_loss: 0.5313 - val_acc: 0.8106
-
計算
+關(guān)注
關(guān)注
2文章
451瀏覽量
38847 -
矩陣
+關(guān)注
關(guān)注
0文章
425瀏覽量
34605 -
機制
+關(guān)注
關(guān)注
0文章
24瀏覽量
9813
原文標(biāo)題:機器如何讀懂人心:Keras實現(xiàn)Self-Attention文本分類
文章出處:【微信號:rgznai100,微信公眾號:rgznai100】歡迎添加關(guān)注!文章轉(zhuǎn)載請注明出處。
發(fā)布評論請先 登錄
相關(guān)推薦
面向列的HBase存儲結(jié)構(gòu)究竟有什么樣的不同之處呢?
暴風(fēng)電視拆機圖解 內(nèi)部結(jié)構(gòu)究竟是怎樣的
BERT模型的PyTorch實現(xiàn)
為什么要有attention機制,Attention原理
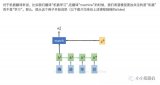
AAAI 2019 Gaussian Transformer 一種自然語言推理方法
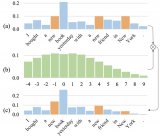
解析Transformer中的位置編碼 -- ICLR 2021
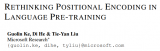
一個LSTM被分解成垂直和水平的LSTM
全球首個面向遙感任務(wù)設(shè)計的億級視覺Transformer大模型
基于視覺transformer的高效時空特征學(xué)習(xí)算法
簡述深度學(xué)習(xí)中的Attention機制
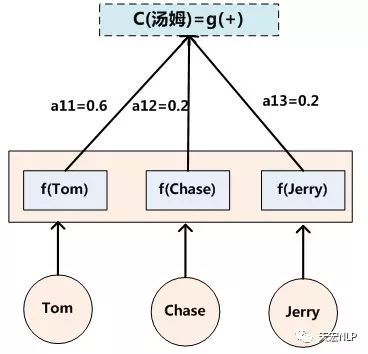
解析ChatGPT背后的技術(shù)演進
如何入門面向自動駕駛領(lǐng)域的視覺Transformer?
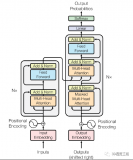
基于Transformer的目標(biāo)檢測算法的3個難點
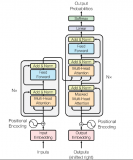
基于Transformer的目標(biāo)檢測算法難點
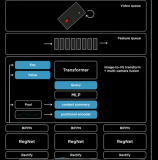
視覺Transformer基本原理及目標(biāo)檢測應(yīng)用
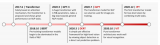
評論