前言
代碼倉庫地址:https://github.com/Oneflow-Inc/one-yolov5歡迎star one-yolov5項目 獲取最新的動態(tài)。如果您有問題,歡迎在倉庫給我們提出寶貴的意見。如果對您有幫助,歡迎來給我Star呀~
源碼解讀: train.py 本文涉及到了大量的超鏈接,但是在微信文章里面外鏈接會被吃掉 ,所以歡迎大家到這里查看本篇文章的完整版本。
這個文件是yolov5的訓(xùn)練腳本。總體代碼流程:
準(zhǔn)備工作: 數(shù)據(jù) + 模型 + 學(xué)習(xí)率 + 優(yōu)化器
訓(xùn)練過程:
一個訓(xùn)練過程(不包括數(shù)據(jù)準(zhǔn)備),會輪詢多次訓(xùn)練集,每次稱為一個epoch,每個epoch又分為多個batch來訓(xùn)練。流程先后拆解成:
開始訓(xùn)練
訓(xùn)練一個epoch前
訓(xùn)練一個batch前
訓(xùn)練一個batch后
訓(xùn)練一個epoch后。
評估驗證集
結(jié)束訓(xùn)練
1. 導(dǎo)入需要的包和基本配置
importargparse#解析命令行參數(shù)模塊 importmath#數(shù)學(xué)公式模塊 importos#與操作系統(tǒng)進(jìn)行交互的模塊包含文件路徑操作和解析 importrandom#生成隨機數(shù)的模塊 importsys#sys系統(tǒng)模塊包含了與Python解釋器和它的環(huán)境有關(guān)的函數(shù) importtime#時間模塊更底層 fromcopyimportdeepcopy#深拷貝模塊 fromdatetimeimportdatetime#基本日期和時間類型模塊 frompathlibimportPath#Path模塊將str轉(zhuǎn)換為Path對象使字符串路徑易于操作 importnumpyasnp#numpy數(shù)組操作模塊 importoneflowasflow#OneFlow深度學(xué)習(xí)框架 importoneflow.distributedasdist#分布式訓(xùn)練模塊 importoneflow.nnasnn#對oneflow.nn.functional的類的封裝有很多和oneflow.nn.functional相同的函數(shù) importyaml#操作yaml文件模塊 fromoneflow.optimimportlr_scheduler#學(xué)習(xí)率模塊 fromtqdmimporttqdm#進(jìn)度條模塊 importval#導(dǎo)入val.py,forend-of-epochmAP frommodels.experimentalimportattempt_load#導(dǎo)入在線下載模塊 frommodels.yoloimportModel#導(dǎo)入YOLOv5的模型定義 fromutils.autoanchorimportcheck_anchors#導(dǎo)入檢查anchors合法性的函數(shù) #Callbackshttps://start.oneflow.org/oneflow-yolo-doc/source_code_interpretation/callbacks_py.html fromutils.callbacksimportCallbacks#和日志相關(guān)的回調(diào)函數(shù) #dataloadershttps://github.com/Oneflow-Inc/oneflow-yolo-doc/blob/master/docs/source_code_interpretation/utils/dataladers_py.md fromutils.dataloadersimportcreate_dataloader#加載數(shù)據(jù)集的函數(shù) #downloadshttps://github.com/Oneflow-Inc/oneflow-yolo-doc/blob/master/docs/source_code_interpretation/utils/downloads_py.md fromutils.downloadsimportis_url#判斷當(dāng)前字符串是否是鏈接 #generalhttps://github.com/Oneflow-Inc/oneflow-yolo-doc/blob/master/docs/source_code_interpretation/utils/general_py.md fromutils.generalimportcheck_img_size#check_suffix, fromutils.generalimport( LOGGER, check_dataset, check_file, check_git_status, check_requirements, check_yaml, colorstr, get_latest_run, increment_path, init_seeds, intersect_dicts, labels_to_class_weights, labels_to_image_weights, methods, one_cycle, print_args, print_mutation, strip_optimizer, yaml_save, model_save, ) fromutils.loggersimportLoggers#導(dǎo)入日志管理模塊 fromutils.loggers.wandb.wandb_utilsimportcheck_wandb_resume fromutils.lossimportComputeLoss#導(dǎo)入計算Loss的模塊 #在YOLOv5中,fitness函數(shù)實現(xiàn)對[P,R,mAP@.5,mAP@.5-.95]指標(biāo)進(jìn)行加權(quán) fromutils.metricsimportfitness fromutils.oneflow_utilsimportEarlyStopping,ModelEMA,de_parallel,select_device,smart_DDP,smart_optimizer,smart_resume#導(dǎo)入早停機制模塊,模型滑動平均更新模塊,解分布式模塊,智能選擇設(shè)備,智能優(yōu)化器以及智能斷點續(xù)訓(xùn)模塊等 fromutils.plotsimportplot_evolve,plot_labels #LOCAL_RANK:當(dāng)前進(jìn)程對應(yīng)的GPU號。 LOCAL_RANK=int(os.getenv("LOCAL_RANK",-1))#https://pytorch.org/docs/stable/elastic/run.html #RANK:當(dāng)前進(jìn)程的序號,用于進(jìn)程間通訊,rank=0的主機為master節(jié)點。 RANK=int(os.getenv("RANK",-1)) #WORLD_SIZE:總的進(jìn)程數(shù)量(原則上第一個process占用一個GPU是較優(yōu)的)。 WORLD_SIZE=int(os.getenv("WORLD_SIZE",1)) #Linux下: #FILE='path/to/one-yolov5/train.py' #將'path/to/one-yolov5'加入系統(tǒng)的環(huán)境變量該腳本結(jié)束后失效。 FILE=Path(__file__).resolve() ROOT=FILE.parents[0]#YOLOv5rootdirectory ifstr(ROOT)notinsys.path: sys.path.append(str(ROOT))#addROOTtoPATH ROOT=Path(os.path.relpath(ROOT,Path.cwd()))#relative
2. parse_opt 函數(shù)
這個函數(shù)用于設(shè)置opt參數(shù)
weights:權(quán)重文件 cfg:模型配置文件包括nc、depth_multiple、width_multiple、anchors、backbone、head等 data:數(shù)據(jù)集配置文件包括path、train、val、test、nc、names、download等 hyp:初始超參文件 epochs:訓(xùn)練輪次 batch-size:訓(xùn)練批次大小 img-size:輸入網(wǎng)絡(luò)的圖片分辨率大小 resume:斷點續(xù)訓(xùn),從上次打斷的訓(xùn)練結(jié)果處接著訓(xùn)練默認(rèn)False nosave:不保存模型默認(rèn)False(保存)True:onlytestfinalepoch notest:是否只測試最后一輪默認(rèn)FalseTrue:只測試最后一輪False:每輪訓(xùn)練完都測試mAP workers:dataloader中的最大work數(shù)(線程個數(shù)) device:訓(xùn)練的設(shè)備 single-cls:數(shù)據(jù)集是否只有一個類別默認(rèn)False rect:訓(xùn)練集是否采用矩形訓(xùn)練默認(rèn)False可以參考:https://start.oneflow.org/oneflow-yolo-doc/tutorials/05_chapter/rectangular_reasoning.html noautoanchor:不自動調(diào)整anchor默認(rèn)False(自動調(diào)整anchor) evolve:是否進(jìn)行超參進(jìn)化默認(rèn)False multi-scale:是否使用多尺度訓(xùn)練默認(rèn)False label-smoothing:標(biāo)簽平滑增強默認(rèn)0.0不增強要增強一般就設(shè)為0.1 adam:是否使用adam優(yōu)化器默認(rèn)False(使用SGD) sync-bn:是否使用跨卡同步BN操作,在DDP中使用默認(rèn)False linear-lr:是否使用linearlr線性學(xué)習(xí)率默認(rèn)False使用cosinelr cache-image:是否提前緩存圖片到內(nèi)存cache,以加速訓(xùn)練默認(rèn)False image-weights:是否使用圖片加權(quán)選擇策略(selectionimgtotrainingbyclassweights)默認(rèn)False不使用 bucket:谷歌云盤bucket一般用不到 project:訓(xùn)練結(jié)果保存的根目錄默認(rèn)是runs/train name:訓(xùn)練結(jié)果保存的目錄默認(rèn)是exp最終:runs/train/exp exist-ok:如果文件存在就ok不存在就新建或incrementname默認(rèn)False(默認(rèn)文件都是不存在的) quad:dataloader取數(shù)據(jù)時,是否使用collate_fn4代替collate_fn默認(rèn)False save_period:Logmodelafterevery"save_period"epoch,默認(rèn)-1不需要logmodel信息 artifact_alias:whichversionofdatasetartifacttobestripped默認(rèn)lastest貌似沒用到這個參數(shù)? local_rank:當(dāng)前進(jìn)程對應(yīng)的GPU號。-1且gpu=1時不進(jìn)行分布式 entity:wandbentity默認(rèn)None upload_dataset:是否上傳dataset到wandbtabel(將數(shù)據(jù)集作為交互式dsviz表在瀏覽器中查看、查詢、篩選和分析數(shù)據(jù)集)默認(rèn)False bbox_interval:設(shè)置帶邊界框圖像記錄間隔Setbounding-boximageloggingintervalforW&B默認(rèn)-1opt.epochs//10 bbox_iou_optim:這個參數(shù)代表啟用oneflow針對bbox_iou部分的優(yōu)化,使得訓(xùn)練速度更快
更多細(xì)節(jié)請點這
3 main函數(shù)
3.1 Checks
defmain(opt,callbacks=Callbacks()): #Checks ifRANKin{-1,0}: #輸出所有訓(xùn)練opt參數(shù)train:... print_args(vars(opt)) #檢查代碼版本是否是最新的github:... check_git_status() #檢查requirements.txt所需包是否都滿足requirements:... check_requirements(exclude=["thop"])
3.2 Resume
判斷是否使用斷點續(xù)訓(xùn)resume, 讀取參數(shù)
使用斷點續(xù)訓(xùn) 就從path/to/last模型文件夾中讀取相關(guān)參數(shù);不使用斷點續(xù)訓(xùn) 就從文件中讀取相關(guān)參數(shù)
#2、判斷是否使用斷點續(xù)訓(xùn)resume,讀取參數(shù) ifopt.resumeandnot(check_wandb_resume(opt)oropt.evolve):#resumefromspecifiedormostrecentlast #使用斷點續(xù)訓(xùn)就從last模型文件夾中讀取相關(guān)參數(shù) #如果resume是str,則表示傳入的是模型的路徑地址 #如果resume是True,則通過get_lastest_run()函數(shù)找到runs文件夾中最近的權(quán)重文件last last=Path(check_file(opt.resume)ifisinstance(opt.resume,str)elseget_latest_run()) opt_yaml=last.parent.parent/"opt.yaml"#trainoptionsyaml opt_data=opt.data#originaldataset ifopt_yaml.is_file(): #相關(guān)的opt參數(shù)也要替換成last中的opt參數(shù) withopen(opt_yaml,errors="ignore")asf: d=yaml.safe_load(f) else: d=flow.load(last,map_location="cpu")["opt"] opt=argparse.Namespace(**d)#replace opt.cfg,opt.weights,opt.resume="",str(last),True#reinstate ifis_url(opt_data): opt.data=check_file(opt_data)#avoidHUBresumeauthtimeout else: #不使用斷點續(xù)訓(xùn)就從文件中讀取相關(guān)參數(shù) #opt.hyp=opt.hypor('hyp.finetune.yaml'ifopt.weightselse'hyp.scratch.yaml') opt.data,opt.cfg,opt.hyp,opt.weights,opt.project=( check_file(opt.data), check_yaml(opt.cfg), check_yaml(opt.hyp), str(opt.weights), str(opt.project), )#checks assertlen(opt.cfg)orlen(opt.weights),"either--cfgor--weightsmustbespecified" ifopt.evolve: ifopt.project==str(ROOT/"runs/train"):#ifdefaultprojectname,renametoruns/evolve opt.project=str(ROOT/"runs/evolve") opt.exist_ok,opt.resume=( opt.resume, False, )#passresumetoexist_okanddisableresume ifopt.name=="cfg": opt.name=Path(opt.cfg).stem#usemodel.yamlasname #根據(jù)opt.project生成目錄如:runs/train/exp18 opt.save_dir=str(increment_path(Path(opt.project)/opt.name,exist_ok=opt.exist_ok))
3.3 DDP mode
DDP mode設(shè)置
#3、DDP模式的設(shè)置 """select_device select_device函數(shù):設(shè)置當(dāng)前腳本的device:cpu或者cuda。 并且當(dāng)且僅當(dāng)使用cuda時并且有多塊gpu時可以使用ddp模式,否則拋出報錯信息。batch_size需要整除總的進(jìn)程數(shù)量。 另外DDP模式不支持AutoBatch功能,使用DDP模式必須手動指定batchsize。 """ device=select_device(opt.device,batch_size=opt.batch_size) ifLOCAL_RANK!=-1: msg="isnotcompatiblewithYOLOv5Multi-GPUDDPtraining" assertnotopt.image_weights,f"--image-weights{msg}" assertnotopt.evolve,f"--evolve{msg}" assertopt.batch_size!=-1,f"AutoBatchwith--batch-size-1{msg},pleasepassavalid--batch-size" assertopt.batch_size%WORLD_SIZE==0,f"--batch-size{opt.batch_size}mustbemultipleofWORLD_SIZE" assertflow.cuda.device_count()>LOCAL_RANK,"insufficientCUDAdevicesforDDPcommand" flow.cuda.set_device(LOCAL_RANK) device=flow.device("cuda",LOCAL_RANK)
3.4Train
不使用進(jìn)化算法 正常Train
#Train ifnotopt.evolve: #如果不進(jìn)行超參進(jìn)化那么就直接調(diào)用train()函數(shù),開始訓(xùn)練 train(opt.hyp,opt,device,callbacks)
3.5 Evolve hyperparameters (optional)
遺傳進(jìn)化算法,先進(jìn)化出最佳超參后訓(xùn)練
#否則使用超參進(jìn)化算法(遺傳算法)求出最佳超參再進(jìn)行訓(xùn)練 else: #Hyperparameterevolutionmetadata(mutationscale0-1,lower_limit,upper_limit) #超參進(jìn)化列表(突變規(guī)模,最小值,最大值) meta={ "lr0":(1,1e-5,1e-1),#initiallearningrate(SGD=1E-2,Adam=1E-3) "lrf":(1,0.01,1.0),#finalOneCycleLRlearningrate(lr0*lrf) "momentum":(0.3,0.6,0.98),#SGDmomentum/Adambeta1 "weight_decay":(1,0.0,0.001),#optimizerweightdecay "warmup_epochs":(1,0.0,5.0),#warmupepochs(fractionsok) "warmup_momentum":(1,0.0,0.95),#warmupinitialmomentum "warmup_bias_lr":(1,0.0,0.2),#warmupinitialbiaslr "box":(1,0.02,0.2),#boxlossgain "cls":(1,0.2,4.0),#clslossgain "cls_pw":(1,0.5,2.0),#clsBCELosspositive_weight "obj":(1,0.2,4.0),#objlossgain(scalewithpixels) "obj_pw":(1,0.5,2.0),#objBCELosspositive_weight "iou_t":(0,0.1,0.7),#IoUtrainingthreshold "anchor_t":(1,2.0,8.0),#anchor-multiplethreshold "anchors":(2,2.0,10.0),#anchorsperoutputgrid(0toignore) "fl_gamma":(0,0.0,2.0),#focallossgamma(efficientDetdefaultgamma=1.5) "hsv_h":(1,0.0,0.1),#imageHSV-Hueaugmentation(fraction) "hsv_s":(1,0.0,0.9),#imageHSV-Saturationaugmentation(fraction) "hsv_v":(1,0.0,0.9),#imageHSV-Valueaugmentation(fraction) "degrees":(1,0.0,45.0),#imagerotation(+/-deg) "translate":(1,0.0,0.9),#imagetranslation(+/-fraction) "scale":(1,0.0,0.9),#imagescale(+/-gain) "shear":(1,0.0,10.0),#imageshear(+/-deg) "perspective":(0,0.0,0.001),#imageperspective(+/-fraction),range0-0.001 "flipud":(1,0.0,1.0),#imageflipup-down(probability) "fliplr":(0,0.0,1.0),#imageflipleft-right(probability) "mosaic":(1,0.0,1.0),#imagemixup(probability) "mixup":(1,0.0,1.0),#imagemixup(probability) "copy_paste":(1,0.0,1.0), }#segmentcopy-paste(probability) withopen(opt.hyp,errors="ignore")asf:#載入初始超參 hyp=yaml.safe_load(f)#loadhypsdict if"anchors"notinhyp:#anchorscommentedinhyp.yaml hyp["anchors"]=3 opt.noval,opt.nosave,save_dir=( True, True, Path(opt.save_dir), )#onlyval/savefinalepoch #ei=[isinstance(x,(int,float))forxinhyp.values()]#evolvableindices #evolve_yaml超參進(jìn)化后文件保存地址 evolve_yaml,evolve_csv=save_dir/"hyp_evolve.yaml",save_dir/"evolve.csv" ifopt.bucket: os.system(f"gsutilcpgs://{opt.bucket}/evolve.csv{evolve_csv}")#downloadevolve.csvifexists """ 使用遺傳算法進(jìn)行參數(shù)進(jìn)化默認(rèn)是進(jìn)化300代 這里的進(jìn)化算法原理為:根據(jù)之前訓(xùn)練時的hyp來確定一個basehyp再進(jìn)行突變,具體是通過之前每次進(jìn)化得到的results來確定之前每個hyp的權(quán)重,有了每個hyp和每個hyp的權(quán)重之后有兩種進(jìn)化方式; 1.根據(jù)每個hyp的權(quán)重隨機選擇一個之前的hyp作為basehyp,random.choices(range(n),weights=w) 2.根據(jù)每個hyp的權(quán)重對之前所有的hyp進(jìn)行融合獲得一個basehyp,(x*w.reshape(n,1)).sum(0)/w.sum() evolve.txt會記錄每次進(jìn)化之后的results+hyp 每次進(jìn)化時,hyp會根據(jù)之前的results進(jìn)行從大到小的排序; 再根據(jù)fitness函數(shù)計算之前每次進(jìn)化得到的hyp的權(quán)重 (其中fitness是我們尋求最大化的值。在YOLOv5中,fitness函數(shù)實現(xiàn)對[P,R,mAP@.5,mAP@.5-.95]指標(biāo)進(jìn)行加權(quán)。) 再確定哪一種進(jìn)化方式,從而進(jìn)行進(jìn)化。 這部分代碼其實不是很重要并且也比較難理解,大家如果沒有特殊必要的話可以忽略,因為正常訓(xùn)練也不會用到超參數(shù)進(jìn)化。 """ for_inrange(opt.evolve):#generationstoevolve ifevolve_csv.exists():#ifevolve.csvexists:selectbesthypsandmutate #Selectparent(s) parent="single"#parentselectionmethod:'single'or'weighted' x=np.loadtxt(evolve_csv,ndmin=2,delimiter=",",skiprows=1) n=min(5,len(x))#numberofpreviousresultstoconsider #fitness是我們尋求最大化的值。在YOLOv5中,fitness函數(shù)實現(xiàn)對[P,R,mAP@.5,mAP@.5-.95]指標(biāo)進(jìn)行加權(quán) x=x[np.argsort(-fitness(x))][:n]#topnmutations w=fitness(x)-fitness(x).min()+1e-6#weights(sum>0) ifparent=="single"orlen(x)==1: #x=x[random.randint(0,n-1)]#randomselection x=x[random.choices(range(n),weights=w)[0]]#weightedselection elifparent=="weighted": x=(x*w.reshape(n,1)).sum(0)/w.sum()#weightedcombination #Mutate mp,s=0.8,0.2#mutationprobability,sigma npr=np.random npr.seed(int(time.time())) g=np.array([meta[k][0]forkinhyp.keys()])#gains0-1 ng=len(meta) v=np.ones(ng) whileall(v==1):#mutateuntilachangeoccurs(preventduplicates) v=(g*(npr.random(ng)
4 def train(hyp, opt, device, callbacks):
4.1 載入?yún)?shù)
""" :paramshyp:data/hyps/hyp.scratch.yamlhypdictionary :paramsopt:main中opt參數(shù) :paramsdevice:當(dāng)前設(shè)備 :paramscallbacks:和日志相關(guān)的回調(diào)函數(shù)https://start.oneflow.org/oneflow-yolo-doc/source_code_interpretation/callbacks_py.html """ deftrain(hyp,opt,device,callbacks):#hypispath/to/hyp.yamlorhypdictionary (save_dir,epochs,batch_size,weights,single_cls,evolve,data,cfg,resume,noval,nosave,workers,freeze,bbox_iou_optim)=( Path(opt.save_dir), opt.epochs, opt.batch_size, opt.weights, opt.single_cls, opt.evolve, opt.data, opt.cfg, opt.resume, opt.noval, opt.nosave, opt.workers, opt.freeze, opt.bbox_iou_optim, )
4.2 初始化參數(shù)和配置信息
下面輸出超參數(shù)的時候截圖如下:
#和日志相關(guān)的回調(diào)函數(shù),記錄當(dāng)前代碼執(zhí)行的階段 callbacks.run("on_pretrain_routine_start") #保存權(quán)重路徑如runs/train/exp18/weights w=save_dir/"weights"#weightsdir (w.parentifevolveelsew).mkdir(parents=True,exist_ok=True)#makedir last,best=w/"last",w/"best" #Hyperparameters超參 ifisinstance(hyp,str): withopen(hyp,errors="ignore")asf: #loadhypsdict加載超參信息 hyp=yaml.safe_load(f)#loadhypsdict #日志輸出超參信息hyperparameters:... LOGGER.info(colorstr("hyperparameters:")+",".join(f"{k}={v}"fork,vinhyp.items())) opt.hyp=hyp.copy()#forsavinghypstocheckpoints #保存運行時的參數(shù)配置 ifnotevolve: yaml_save(save_dir/"hyp.yaml",hyp) yaml_save(save_dir/"opt.yaml",vars(opt)) #Loggers data_dict=None ifRANKin{-1,0}: #初始化Loggers對象 #def__init__(self,save_dir=None,weights=None,opt=None,hyp=None,logger=None,include=LOGGERS): loggers=Loggers(save_dir,weights,opt,hyp,LOGGER)#loggersinstance #Registeractions forkinmethods(loggers):#注冊鉤子https://github.com/Oneflow-Inc/one-yolov5/blob/main/utils/callbacks.py callbacks.register_action(k,callback=getattr(loggers,k)) #Config #是否需要畫圖:所有的labels信息、迭代的epochs、訓(xùn)練結(jié)果等 plots=notevolveandnotopt.noplots#createplots cuda=device.type!="cpu" #初始化隨機數(shù)種子 init_seeds(opt.seed+1+RANK,deterministic=True) data_dict=data_dictorcheck_dataset(data)#checkifNone train_path,val_path=data_dict["train"],data_dict["val"] #nc:numberofclasses數(shù)據(jù)集有多少種類別 nc=1ifsingle_clselseint(data_dict["nc"])#numberofclasses #如果只有一個類別并且data_dict里沒有names這個key的話,我們將names設(shè)置為["item"]代表目標(biāo) names=["item"]ifsingle_clsandlen(data_dict["names"])!=1elsedata_dict["names"]#classnames assertlen(names)==nc,f"{len(names)}namesfoundfornc={nc}datasetin{data}"#check #當(dāng)前數(shù)據(jù)集是否是coco數(shù)據(jù)集(80個類別) is_coco=isinstance(val_path,str)andval_path.endswith("coco/val2017.txt")#COCOdataset
4.3 model
#檢查權(quán)重命名合法性: #合法:pretrained=True; #不合法:pretrained=False; pretrained=check_wights(weights) #載入模型 ifpretrained: #使用預(yù)訓(xùn)練 #---------------------------------------------------------# #加載模型及參數(shù) ckpt=flow.load(weights,map_location="cpu")#loadcheckpointtoCPUtoavoidCUDAmemoryleak #這里加載模型有兩種方式,一種是通過opt.cfg另一種是通過ckpt['model'].yaml #區(qū)別在于是否使用resume如果使用resume會將opt.cfg設(shè)為空,按照ckpt['model'].yaml來創(chuàng)建模型 #這也影響了下面是否除去anchor的key(也就是不加載anchor),如果resume則不加載anchor #原因:保存的模型會保存anchors,有時候用戶自定義了anchor之后,再resume,則原來基于coco數(shù)據(jù)集的anchor會自己覆蓋自己設(shè)定的anchor #詳情參考:https://github.com/ultralytics/yolov5/issues/459 #所以下面設(shè)置intersect_dicts()就是忽略exclude model=Model(cfgorckpt["model"].yaml,ch=3,nc=nc,anchors=hyp.get("anchors")).to(device)#create exclude=["anchor"]if(cfgorhyp.get("anchors"))andnotresumeelse[]#excludekeys csd=ckpt["model"].float().state_dict()#checkpointstate_dictasFP32 #篩選字典中的鍵值對把exclude刪除 csd=intersect_dicts(csd,model.state_dict(),exclude=exclude)#intersect #載入模型權(quán)重 model.load_state_dict(csd,strict=False)#load LOGGER.info(f"Transferred{len(csd)}/{len(model.state_dict())}itemsfrom{weights}")#report else: #不使用預(yù)訓(xùn)練 model=Model(cfg,ch=3,nc=nc,anchors=hyp.get("anchors")).to(device)#create #注意一下:one-yolov5的amp訓(xùn)練還在開發(fā)調(diào)試中,暫時關(guān)閉,后續(xù)支持后打開。但half的推理目前我們是支持的 #amp=check_amp(model)#checkAMP amp=False #Freeze #凍結(jié)權(quán)重層 #這里只是給了凍結(jié)權(quán)重層的一個例子,但是作者并不建議凍結(jié)權(quán)重層,訓(xùn)練全部層參數(shù),可以得到更好的性能,不過也會更慢 freeze=[f"model.{x}."forxin(freezeiflen(freeze)>1elserange(freeze[0]))]#layerstofreeze fork,vinmodel.named_parameters(): v.requires_grad=True#trainalllayers #NaNto0(commentedforerratictrainingresults) #v.register_hook(lambdax:torch.nan_to_num(x)) ifany(xinkforxinfreeze): LOGGER.info(f"freezing{k}") v.requires_grad=False
4.4 Optimizer
選擇優(yōu)化器
#Optimizer nbs=64#nominalbatchsize accumulate=max(round(nbs/batch_size),1)#accumulatelossbeforeoptimizing hyp["weight_decay"]*=batch_size*accumulate/nbs#scaleweight_decay optimizer=smart_optimizer(model,opt.optimizer,hyp["lr0"],hyp["momentum"],hyp["weight_decay"])
4.5 學(xué)習(xí)率
#Scheduler ifopt.cos_lr: #使用onecycle學(xué)習(xí)率https://arxiv.org/pdf/1803.09820.pdf lf=one_cycle(1,hyp["lrf"],epochs)#cosine1->hyp['lrf'] else: #使用線性學(xué)習(xí)率 deff(x): return(1-x/epochs)*(1.0-hyp["lrf"])+hyp["lrf"] lf=f#linear #實例化scheduler scheduler=lr_scheduler.LambdaLR(optimizer,lr_lambda=lf)#plot_lr_scheduler(optimizer,scheduler,epochs)
4.6 EMA
單卡訓(xùn)練: 使用EMA(指數(shù)移動平均)對模型的參數(shù)做平均, 一種給予近期數(shù)據(jù)更高權(quán)重的平均方法, 以求提高測試指標(biāo)并增加模型魯棒。
#EMA ema=ModelEMA(model)ifRANKin{-1,0}elseNone
4.7 Resume
斷點續(xù)訓(xùn)
#Resume best_fitness,start_epoch=0.0,0 ifpretrained: ifresume: best_fitness,start_epoch,epochs=smart_resume(ckpt,optimizer,ema,weights,epochs,resume) delckpt,csd
4.8 SyncBatchNorm
SyncBatchNorm可以提高多gpu訓(xùn)練的準(zhǔn)確性,但會顯著降低訓(xùn)練速度。它僅適用于多GPU DistributedDataParallel 訓(xùn)練。
#SyncBatchNorm ifopt.sync_bnandcudaandRANK!=-1: model=flow.nn.SyncBatchNorm.convert_sync_batchnorm(model).to(device) LOGGER.info("UsingSyncBatchNorm()")
4.9 數(shù)據(jù)加載
#Trainloaderhttps://start.oneflow.org/oneflow-yolo-doc/source_code_interpretation/utils/dataladers_py.html train_loader,dataset=create_dataloader( train_path, imgsz, batch_size//WORLD_SIZE, gs, single_cls, hyp=hyp, augment=True, cache=Noneifopt.cache=="val"elseopt.cache, rect=opt.rect, rank=LOCAL_RANK, workers=workers, image_weights=opt.image_weights, quad=opt.quad, prefix=colorstr("train:"), shuffle=True, ) labels=np.concatenate(dataset.labels,0) #獲取標(biāo)簽中最大類別值,與類別數(shù)作比較,如果大于等于類別數(shù)則表示有問題 mlc=int(labels[:,0].max())#maxlabelclass assertmlc
4.10 DDP mode
#DDPmode ifcudaandRANK!=-1: model=smart_DDP(model)
4.11 附加model attributes
#Modelattributes nl=de_parallel(model).model[-1].nl#numberofdetectionlayers(toscalehyps) hyp["box"]*=3/nl#scaletolayers hyp["cls"]*=nc/80*3/nl#scaletoclassesandlayers hyp["obj"]*=(imgsz/640)**2*3/nl#scaletoimagesizeandlayers hyp["label_smoothing"]=opt.label_smoothing model.nc=nc#attachnumberofclassestomodel model.hyp=hyp#attachhyperparameterstomodel #從訓(xùn)練樣本標(biāo)簽得到類別權(quán)重(和類別中的目標(biāo)數(shù)即類別頻率成反比) model.class_weights=labels_to_class_weights(dataset.labels,nc).to(device)*nc#attachclassweights model.names=names#獲取類別名
4.12 Start training
#Starttraining t0=time.time() nb=len(train_loader)#numberofbatches #獲取預(yù)熱迭代的次數(shù)iterations#numberofwarmupiterations,max(3epochs,1kiterations) nw=max(round(hyp["warmup_epochs"]*nb),100)#numberofwarmupiterations,max(3epochs,100iterations) #nw=min(nw,(epochs-start_epoch)/2*nb)#limitwarmupto1/2?of?training last_opt_step?=?-1 #?初始化maps(每個類別的map)和results maps?=?np.zeros(nc)??#?mAP?per?class results?=?(0,?0,?0,?0,?0,?0,?0)??#?P,?R,?mAP@.5,?mAP@.5-.95,?val_loss(box,?obj,?cls) #?設(shè)置學(xué)習(xí)率衰減所進(jìn)行到的輪次,即使打斷訓(xùn)練,使用resume接著訓(xùn)練也能正常銜接之前的訓(xùn)練進(jìn)行學(xué)習(xí)率衰減 scheduler.last_epoch?=?start_epoch?-?1??#?do?not?move #?scaler?=?flow.cuda.amp.GradScaler(enabled=amp)?這個是和amp相關(guān)的loss縮放模塊,后續(xù)one-yolv5支持好amp訓(xùn)練后會打開 stopper,?_?=?EarlyStopping(patience=opt.patience),?False #?初始化損失函數(shù) #?這里的bbox_iou_optim是one-yolov5擴展的一個參數(shù),可以啟用更快的bbox_iou函數(shù),模型訓(xùn)練速度比PyTorch更快。 compute_loss?=?ComputeLoss(model,?bbox_iou_optim=bbox_iou_optim)??#?init?loss?class callbacks.run("on_train_start") #?打印日志信息 LOGGER.info( ????f"Image?sizes?{imgsz}?train,?{imgsz}?val " ????f"Using?{train_loader.num_workers?*?WORLD_SIZE}?dataloader?workers " ????f"Logging?results?to?{colorstr('bold',?save_dir)} " ????f"Starting?training?for?{epochs}?epochs..." ) for?epoch?in?range(start_epoch,?epochs):??#?epoch?------------------------------------------------------------------ ????callbacks.run("on_train_epoch_start") ????model.train() ????#?Update?image?weights?(optional,?single-GPU?only) ????#?Update?image?weights?(optional)??并不一定好??默認(rèn)是False的 ????#?如果為True?進(jìn)行圖片采樣策略(按數(shù)據(jù)集各類別權(quán)重采樣) ????if?opt.image_weights: ????????#?根據(jù)前面初始化的圖片采樣權(quán)重model.class_weights(每個類別的權(quán)重?頻率高的權(quán)重小)以及maps配合每張圖片包含的類別數(shù) ????????#?通過rando.choices生成圖片索引indices從而進(jìn)行采用?(作者自己寫的采樣策略,效果不一定ok) ????????cw?=?model.class_weights.cpu().numpy()?*?(1?-?maps)?**?2?/?nc??#?class?weights ????????#?labels_to_image_weights:?這個函數(shù)是利用每張圖片真實gt框的真實標(biāo)簽labels和開始訓(xùn)練前通過?labels_to_class_weights函數(shù) ????????#?得到的每個類別的權(quán)重得到數(shù)據(jù)集中每張圖片對應(yīng)的權(quán)重。 ????????#?https://github.com/Oneflow-Inc/oneflow-yolo-doc/blob/master/docs/source_code_interpretation/utils/general_py.md#192-labels_to_image_weights ????????iw?=?labels_to_image_weights(dataset.labels,?nc=nc,?class_weights=cw)??#?image?weights ????????dataset.indices?=?random.choices(range(dataset.n),?weights=iw,?k=dataset.n)??#?rand?weighted?idx ????#?初始化訓(xùn)練時打印的平均損失信息 ????mloss?=?flow.zeros(3,?device=device)??#?mean?losses ????if?RANK?!=?-1: ????????#?DDP模式打亂數(shù)據(jù),并且ddp.sampler的隨機采樣數(shù)據(jù)是基于epoch+seed作為隨機種子,每次epoch不同,隨機種子不同 ????????train_loader.sampler.set_epoch(epoch) ???? ????#?進(jìn)度條,方便展示信息 ????pbar?=?enumerate(train_loader) ????LOGGER.info((' '?+?'%11s'?*?7)?%?('Epoch',?'GPU_mem',?'box_loss',?'obj_loss',?'cls_loss',?'Instances',?'Size')) ????if?RANK?in?{-1,?0}: ????????#?創(chuàng)建進(jìn)度條 ????????pbar?=?tqdm(pbar,?total=nb,?bar_format="{l_bar}{bar:10}{r_bar}{bar:-10b}")??#?progress?bar ???? ????#?梯度清零 ????optimizer.zero_grad() ????for?i,?( ????????imgs, ????????targets, ????????paths, ????????_, ????)?in?pbar:??#?batch?------------------------------------------------------------- ????????callbacks.run("on_train_batch_start") ????????#?ni:?計算當(dāng)前迭代次數(shù)?iteration ????????ni?=?i?+?nb?*?epoch??#?number?integrated?batches?(since?train?start) ????????imgs?=?imgs.to(device).float()?/?255??#?uint8?to?float32,?0-255?to?0.0-1.0 ????????#?Warmup ????????#?預(yù)熱訓(xùn)練(前nw次迭代)熱身訓(xùn)練迭代的次數(shù)iteration范圍[1:nw]??選取較小的accumulate,學(xué)習(xí)率以及momentum,慢慢的訓(xùn)練 ????????if?ni?<=?nw: ????????????xi?=?[0,?nw]??#?x?interp ????????????#?compute_loss.gr?=?np.interp(ni,?xi,?[0.0,?1.0])??#?iou?loss?ratio?(obj_loss?=?1.0?or?iou) ????????????accumulate?=?max(1,?np.interp(ni,?xi,?[1,?nbs?/?batch_size]).round()) ????????????for?j,?x?in?enumerate(optimizer.param_groups): ????????????????#?bias?lr?falls?from?0.1?to?lr0,?all?other?lrs?rise?from?0.0?to?lr0 ????????????????x["lr"]?=?np.interp( ????????????????????ni, ????????????????????xi, ????????????????????[hyp["warmup_bias_lr"]?if?j?==?0?else?0.0,?x["initial_lr"]?*?lf(epoch)], ????????????????) ????????????????if?"momentum"?in?x: ????????????????????x["momentum"]?=?np.interp(ni,?xi,?[hyp["warmup_momentum"],?hyp["momentum"]]) ????????#?Multi-scale?默認(rèn)關(guān)閉 ????????#?Multi-scale?多尺度訓(xùn)練???從[imgsz*0.5,?imgsz*1.5+gs]間隨機選取一個尺寸(32的倍數(shù))作為當(dāng)前batch的尺寸送入模型開始訓(xùn)練 ????????#?imgsz:?默認(rèn)訓(xùn)練尺寸???gs:?模型最大stride=32???[32?16?8] ????????if?opt.multi_scale: ????????????sz?=?random.randrange(imgsz?*?0.5,?imgsz?*?1.5?+?gs)?//?gs?*?gs??#?size ????????????sf?=?sz?/?max(imgs.shape[2:])??#?scale?factor ????????????if?sf?!=?1: ????????????????ns?=?[math.ceil(x?*?sf?/?gs)?*?gs?for?x?in?imgs.shape[2:]]??#?new?shape?(stretched?to?gs-multiple) ????????????????#?下采樣 ????????????????imgs?=?nn.functional.interpolate(imgs,?size=ns,?mode="bilinear",?align_corners=False) ????????#?Forward ????????pred?=?model(imgs)??#?forward ????????loss,?loss_items?=?compute_loss(pred,?targets.to(device))??#?loss?scaled?by?batch_size ????????if?RANK?!=?-1: ????????????loss?*=?WORLD_SIZE??#?gradient?averaged?between?devices?in?DDP?mode ????????if?opt.quad: ????????????loss?*=?4.0 ????????#?Backward ????????#?scaler.scale(loss).backward() ????????#?Backward??反向傳播?? ????????loss.backward() ????????#?Optimize?-?https://pytorch.org/docs/master/notes/amp_examples.html ????????#?模型反向傳播accumulate次(iterations)后再根據(jù)累計的梯度更新一次參數(shù) ????????if?ni?-?last_opt_step?>=accumulate: #optimizer.step參數(shù)更新 optimizer.step() #梯度清零 optimizer.zero_grad() ifema: #當(dāng)前epoch訓(xùn)練結(jié)束更新ema ema.update(model) last_opt_step=ni #Log #打印Print一些信息包括當(dāng)前epoch、顯存、損失(box、obj、cls、total)、當(dāng)前batch的target的數(shù)量和圖片的size等信息 ifRANKin{-1,0}: mloss=(mloss*i+loss_items)/(i+1)#updatemeanlosses pbar.set_description(("%11s"+"%11.4g"*5)%(f"{epoch}/{epochs-1}",*mloss,targets.shape[0],imgs.shape[-1])) #endbatch---------------------------------------------------------------- #Scheduler lr=[x["lr"]forxinoptimizer.param_groups]#forloggers scheduler.step() ifRANKin{-1,0}: #mAP callbacks.run("on_train_epoch_end",epoch=epoch) ema.update_attr(model,include=["yaml","nc","hyp","names","stride","class_weights"]) final_epoch=(epoch+1==epochs)orstopper.possible_stop ifnotnovalorfinal_epoch:#CalculatemAP #測試使用的是ema(指數(shù)移動平均對模型的參數(shù)做平均)的模型 #results:[1]Precision所有類別的平均precision(最大f1時) #[1]Recall所有類別的平均recall #[1]map@0.5所有類別的平均mAP@0.5 #[1]map@0.5:0.95所有類別的平均mAP@0.5:0.95 #[1]box_loss驗證集回歸損失,obj_loss驗證集置信度損失,cls_loss驗證集分類損失 #maps:[80]記錄每一個類別的ap值 results,maps,_=val.run( data_dict, batch_size=batch_size//WORLD_SIZE*2, imgsz=imgsz, half=amp, model=ema.ema, single_cls=single_cls, dataloader=val_loader, save_dir=save_dir, plots=False, callbacks=callbacks, compute_loss=compute_loss, ) #UpdatebestmAP #fi是我們尋求最大化的值。在YOLOv5中,fitness函數(shù)實現(xiàn)對[P,R,mAP@.5,mAP@.5-.95]指標(biāo)進(jìn)行加權(quán)。 fi=fitness(np.array(results).reshape(1,-1))#weightedcombinationof[P,R,mAP@.5,mAP@.5-.95] #stop=stopper(epoch=epoch,fitness=fi)#earlystopcheck iffi>best_fitness: best_fitness=fi log_vals=list(mloss)+list(results)+lr callbacks.run("on_fit_epoch_end",log_vals,epoch,best_fitness,fi) #Savemodel if(notnosave)or(final_epochandnotevolve):#ifsave ckpt={ "epoch":epoch, "best_fitness":best_fitness, "model":deepcopy(de_parallel(model)).half(), "ema":deepcopy(ema.ema).half(), "updates":ema.updates, "optimizer":optimizer.state_dict(), "wandb_id":loggers.wandb.wandb_run.idifloggers.wandbelseNone, "opt":vars(opt), "date":datetime.now().isoformat(), } #Savelast,bestanddelete model_save(ckpt,last)#flow.save(ckpt,last) ifbest_fitness==fi: model_save(ckpt,best)#flow.save(ckpt,best) ifopt.save_period>0andepoch%opt.save_period==0: print("isok") model_save(ckpt,w/f"epoch{epoch}")#flow.save(ckpt,w/f"epoch{epoch}") delckpt #Write將測試結(jié)果寫入result.txt中 callbacks.run("on_model_save",last,epoch,final_epoch,best_fitness,fi) #endepoch-------------------------------------------------------------------------- #endtraining---------------------------------------------------------------------------
4.13 End
打印一些信息
日志: 打印訓(xùn)練時間、plots可視化訓(xùn)練結(jié)果results1.png、confusion_matrix.png 以及(‘F1’, ‘PR’, ‘P’, ‘R’)曲線變化 、日志信息
通過調(diào)用val.run() 方法驗證在 coco數(shù)據(jù)集上 模型準(zhǔn)確性 + 釋放顯存
Validate a model's accuracy on COCO val or test-dev datasets. Note that pycocotools metrics may be ~1% better than the equivalent repo metrics, as is visible below, due to slight differences in mAP computation.
ifRANKin{-1,0}: LOGGER.info(f" {epoch-start_epoch+1}epochscompletedin{(time.time()-t0)/3600:.3f}hours") forfinlast,best: iff.exists(): strip_optimizer(f)#stripoptimizers iffisbest: LOGGER.info(f" Validating{f}...") results,_,_=val.run( data_dict, batch_size=batch_size//WORLD_SIZE*2, imgsz=imgsz, model=attempt_load(f,device).half(), iou_thres=0.65ifis_cocoelse0.60,#bestpycocotoolsresultsat0.65 single_cls=single_cls, dataloader=val_loader, save_dir=save_dir, save_json=is_coco, verbose=True, plots=plots, callbacks=callbacks, compute_loss=compute_loss, )#valbestmodelwithplots callbacks.run("on_train_end",last,best,plots,epoch,results) flow.cuda.empty_cache() return
5 run函數(shù)
封裝train接口 支持函數(shù)調(diào)用執(zhí)行這個train.py腳本
defrun(**kwargs): #Usage:importtrain;train.run(data='coco128.yaml',imgsz=320,weights='yolov5m') opt=parse_opt(True) fork,vinkwargs.items(): setattr(opt,k,v)#給opt添加屬性 main(opt) returnopt
6 啟動訓(xùn)練時效果展示
審核編輯:湯梓紅
-
代碼
+關(guān)注
關(guān)注
30文章
4819瀏覽量
68878 -
Batch
+關(guān)注
關(guān)注
0文章
6瀏覽量
7168 -
腳本
+關(guān)注
關(guān)注
1文章
391瀏覽量
14916
原文標(biāo)題:《YOLOv5全面解析教程》九,train.py 逐代碼解析
文章出處:【微信號:GiantPandaCV,微信公眾號:GiantPandaCV】歡迎添加關(guān)注!文章轉(zhuǎn)載請注明出處。
發(fā)布評論請先 登錄
相關(guān)推薦
【YOLOv5】LabVIEW+YOLOv5快速實現(xiàn)實時物體識別(Object Detection)含源碼
龍哥手把手教你學(xué)視覺-深度學(xué)習(xí)YOLOV5篇
怎樣使用PyTorch Hub去加載YOLOv5模型
YOLOv5網(wǎng)絡(luò)結(jié)構(gòu)解析
YOLOv5全面解析教程之目標(biāo)檢測模型精確度評估
使用Yolov5 - i.MX8MP進(jìn)行NPU錯誤檢測是什么原因?
如何YOLOv5測試代碼?
yolov5模型onnx轉(zhuǎn)bmodel無法識別出結(jié)果如何解決?
基于YOLOv5的目標(biāo)檢測文檔進(jìn)行的時候出錯如何解決?
YOLOv5全面解析教程:計算mAP用到的numpy函數(shù)詳解
YOLOv5解析之downloads.py 代碼示例
使用旭日X3派的BPU部署Yolov5
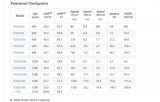
YOLOv8+OpenCV實現(xiàn)DM碼定位檢測與解析
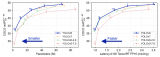
yolov5和YOLOX正負(fù)樣本分配策略
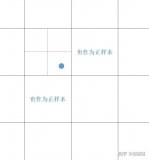
評論