1
背景
近年來(lái),量化感知訓(xùn)練是一個(gè)較為熱點(diǎn)的問(wèn)題,可以大大優(yōu)化量化后訓(xùn)練造成精度損失的問(wèn)題,使得訓(xùn)練過(guò)程更加高效。
Torch.fx在這一問(wèn)題上走在了前列,使用純Python語(yǔ)言實(shí)現(xiàn)了對(duì)于Torch.nn.Module的解析和向IR的轉(zhuǎn)換,也可以提供變換后的IR對(duì)應(yīng)的Python代碼,在外部則是提供了簡(jiǎn)潔易用的API,大大方便了量化感知訓(xùn)練過(guò)程的搭建。此外,Torch.fx也有助于消除動(dòng)態(tài)圖和靜態(tài)圖之間的Gap,可以比較方便地對(duì)圖進(jìn)行操作以及進(jìn)行算子融合。
OneFlow緊隨其后添加了針對(duì)OneFlow的fx,即One-fx,在安裝One-fx之后,用戶可以直接調(diào)用oneflow.fx,也可以直接通過(guò)import onefx as fx進(jìn)行使用。
One-fx實(shí)現(xiàn)代碼中絕大部分是對(duì)于Torch.fx的fork,但根據(jù)OneFlow和PyTorch之間存在的差別進(jìn)行了一些適配或優(yōu)化。本文將圍繞One-fx適配方式以及在OneFlow中的應(yīng)用展開。
2
FX主要模塊
Symbolioc Trace
Graph Module
Interpreter
Proxy
Passes
其中,前4個(gè)模塊共同實(shí)現(xiàn)了fx的基本功能,Graph Module和Proxy又是Symbolic Trace的基礎(chǔ),Passes則是在此基礎(chǔ)上的擴(kuò)充。
Symbolic Trace的基本概念如上圖所示,最基本的模型運(yùn)行過(guò)程就是從模型定義到模型執(zhí)行這樣一個(gè)流程。
fx則是進(jìn)行了非侵入式的解析,將模型執(zhí)行過(guò)程轉(zhuǎn)成一張圖,這張圖中包含了很多個(gè)Node,每一個(gè)Node都包含了模型中的子模塊或者函數(shù)調(diào)用信息,然后用戶可以很方便地獲取到所有的Node,并對(duì)其進(jìn)行一些變換操作,最后通過(guò)GraphModule重新生成一個(gè)模型定義,并對(duì)其執(zhí)行。
其中,在進(jìn)行模型解析的時(shí)候,節(jié)點(diǎn)之間變量傳遞也均使用代理后的變量,如y = oneflow.relu(x),實(shí)際上x和y是Proxy(x)和Proxy(y)。
3
One-fx實(shí)現(xiàn)方式
這里給出一個(gè)Fx最簡(jiǎn)單的用例,以方便后續(xù)對(duì)于實(shí)現(xiàn)方式的介紹。
import oneflow class MyModule(oneflow.nn.Module): def __init__(self): super().__init__() self.linear = oneflow.nn.Linear(512, 512) def forward(self, x): x = self.linear(x) y = oneflow.ones([2, 3]) x = oneflow.relu(x) return y m = MyModule() traced = oneflow.fx.symbolic_trace(m) print(traced.code) """ def forward(self, x): linear = self.linear(x); x = None relu = oneflow.relu(linear); linear = None _tensor_constant0 = self._tensor_constant0 return _tensor_constant0 """?
函數(shù)代理
代理,即fx中的Proxy模塊,目的是在每次進(jìn)行函數(shù)或模塊調(diào)用的時(shí)候添加一些額外操作,使得對(duì)模型的解析和重建得以進(jìn)行,而包裝則是適配代理的一種方式。
torch.fx中,對(duì)于nn.Module的包裝比較易于理解,每當(dāng)待解析Module中出現(xiàn)了繼承自nn.Module的對(duì)象,那么就將其__call__函數(shù)替換成包裝過(guò)的函數(shù)。然而,對(duì)于pytorch的函數(shù)的代理的實(shí)現(xiàn)要更“繞”一些,是借助了__torch_function__這一機(jī)制
限于篇幅原因這里不專門對(duì)其進(jìn)行介紹。比較關(guān)鍵的點(diǎn)是,OneFlow中沒有這一機(jī)制,如果需要添加,那么會(huì)是規(guī)模很大的、侵入性的,于是One-fx的實(shí)現(xiàn)就需要找其它路徑。
我們使用的解決方式是搜索oneflow,oneflow.nn.functional,oneflow._C等模塊中的Callable,并去除其中屬于類的部分,然后對(duì)其余函數(shù)進(jìn)行包裝,在每次解析模型之前,會(huì)將這些模塊的__dict__中對(duì)應(yīng)項(xiàng)替換成包裝后的函數(shù),并且在解析模型之后重新將這些項(xiàng)進(jìn)行還原。對(duì)于constructor類型的函數(shù),如ones,randn等則不進(jìn)行代理,直接運(yùn)行,在最終構(gòu)建圖的時(shí)候作為constant來(lái)處理。
對(duì)于函數(shù)的包裝部分源碼實(shí)現(xiàn)如下,每次運(yùn)行代理后的函數(shù),會(huì)先判斷該函數(shù)的入?yún)⒅杏袥]有Proxy變量,如果有,那么將會(huì)創(chuàng)建一個(gè)call_function類型的節(jié)點(diǎn)并返回Proxy包裝后的節(jié)點(diǎn),否則直接調(diào)用原函數(shù)并返回結(jié)果。
def _create_wrapped_func(orig_fn): @functools.wraps(orig_fn) def wrapped(*args, **kwargs): # 判斷參數(shù)中是否存在proxy變量 proxy = _find_proxy(args, kwargs) if proxy is not None: # 如果參數(shù)中有Proxy變量,創(chuàng)建節(jié)點(diǎn)并返回Proxy包裝后的節(jié)點(diǎn) return_proxy = proxy.tracer.create_proxy( "call_function", orig_fn, args, kwargs ) return_proxy.node.meta["is_wrapped"] = True return return_proxy # 如果沒有Proxy變量,直接調(diào)用原函數(shù) return orig_fn(*args, **kwargs) return wrapped
其中,return_proxy = proxy.tracer.create_proxy("call_function", orig_fn, args, kwargs)這行代碼指定了使用與入?yún)⑾嗤腡racer來(lái)創(chuàng)建節(jié)點(diǎn)并返回結(jié)果,create_proxy函數(shù)定義的主要部分如下,創(chuàng)建節(jié)點(diǎn)并在Proxy包裝后返回。
def create_proxy(self, kind: str, target: Target, args: Tuple[Any, ...], kwargs: Dict[str, Any], name: Optional[str] = None, type_expr : Optional[Any] = None, proxy_factory_fn: Callable[[Node], 'Proxy'] = None): args_ = self.create_arg(args) kwargs_ = self.create_arg(kwargs) assert isinstance(args_, tuple) assert isinstance(kwargs_, dict) # 創(chuàng)建節(jié)點(diǎn) node = self.create_node(kind, target, args_, kwargs_, name, type_expr) if not proxy_factory_fn: proxy = self.proxy(node) else: proxy = proxy_factory_fn(node) return proxy
而其中的create_node方法,實(shí)際上是調(diào)用了Tracer.graph.create_node,在圖中創(chuàng)建節(jié)點(diǎn),主要部分代碼如下,其中op就是fx IR中的op,代表了節(jié)點(diǎn)類型,而target則是節(jié)點(diǎn)的操作主體,在上面的例子中就是orig_func。
因此,當(dāng)我們自定義的Module中的forward函數(shù)中的所有調(diào)用都被包裝之后,實(shí)際上再運(yùn)行forward的時(shí)候,就會(huì)依次在Tracer.graph中創(chuàng)建節(jié)點(diǎn),這也正是symbolic_trace的基本思路。
def create_node(self, op: str, target: 'Target', args: Optional[Tuple['Argument', ...]] = None, kwargs: Optional[Dict[str, 'Argument']] = None, name: Optional[str] = None, type_expr: Optional[Any] = None) -> Node: # 此處有一些assert # 創(chuàng)建一個(gè)節(jié)點(diǎn)名稱,避免重復(fù) candidate = name if name is not None else self._target_to_str(target) name = self._graph_namespace.create_name(candidate, None) # 創(chuàng)建節(jié)點(diǎn) n = Node(self, name, op, target, args, kwargs, type_expr) # 建立名稱與節(jié)點(diǎn)的映射關(guān)系 self._graph_namespace.associate_name_with_obj(name, n) return n
而對(duì)于symbolic_trace過(guò)程,其核心就是Tracer.trace。這個(gè)方法可以分為兩部分,一個(gè)是預(yù)處理部分,一個(gè)是主干部分。其中預(yù)處理過(guò)程大致定義如下,主要任務(wù)是初始化Graph、確立模型以及forward函數(shù)和創(chuàng)建包裝后的參數(shù)。
如前面所提及的,symbolic trace的基本思路是借助Proxy變量以及包裝后的函數(shù),在每次調(diào)用的時(shí)候都創(chuàng)建一個(gè)節(jié)點(diǎn),因此,forward函數(shù)的輸入也需要用Proxy進(jìn)行包裝,這一步定義在Tracer.create_args_for_root中。
?
def trace( self, root: Union[oneflow.nn.Module, Callable[..., Any]], concrete_args: Optional[Dict[str, Any]] = None, ) -> Graph: # 確定模塊主體以及forward函數(shù),其中fn即forward函數(shù) if isinstance(root, oneflow.nn.Module): self.root = root assert hasattr( type(root), self.traced_func_name ), f"traced_func_name={self.traced_func_name} doesn't exist in {type(root).__name__}" fn = getattr(type(root), self.traced_func_name) self.submodule_paths = {mod: name for name, mod in root.named_modules()} else: self.root = oneflow.nn.Module() fn = root tracer_cls: Optional[Type["Tracer"]] = getattr(self, "__class__", None) # 在Tracer中初始化一張圖 self.graph = Graph(tracer_cls=tracer_cls) self.tensor_attrs: Dict[oneflow.Tensor, str] = {} # 這個(gè)子函數(shù)用于收集模型中所有Tensor類型的變量 def collect_tensor_attrs(m: oneflow.nn.Module, prefix_atoms: List[str]): for k, v in m.__dict__.items(): if isinstance(v, oneflow.Tensor): self.tensor_attrs[v] = ".".join(prefix_atoms + [k]) for k, v in m.named_children(): collect_tensor_attrs(v, prefix_atoms + [k]) collect_tensor_attrs(self.root, []) assert isinstance(fn, FunctionType) # 獲取fn所在模塊的所有可讀變量 fn_globals = fn.__globals__ # 創(chuàng)建包裝后的參數(shù) fn, args = self.create_args_for_root( fn, isinstance(root, oneflow.nn.Module), concrete_args )
隨后則是trace的主干部分,這一部分大致代碼如下,主要任務(wù)是對(duì)函數(shù)、方法、模塊進(jìn)行必要的包裝,然后在Graph中創(chuàng)建節(jié)點(diǎn),完成整個(gè)圖的信息。
其中,我們會(huì)創(chuàng)建一個(gè)Patcher環(huán)境并在其中進(jìn)行這些過(guò)程,這是因?yàn)閷?duì)于函數(shù)和方法的包裝會(huì)直接改變掉某些包中對(duì)應(yīng)函數(shù)或方法的行為,為了不讓這種行為的改變溢出到trace的范圍之外,在每次進(jìn)行包裝的時(shí)候會(huì)在Patcher中記錄本次操作,然后在_Patcher.__exit__中根據(jù)記錄的操作一一還原現(xiàn)場(chǎng)。
# 下面代碼仍然是`trace`函數(shù)的一部分 # 定義對(duì)于`nn.Module`的getattr方法的包裝 @functools.wraps(_orig_module_getattr) def module_getattr_wrapper(mod, attr): attr_val = _orig_module_getattr(mod, attr) return self.getattr(attr, attr_val, parameter_proxy_cache) # 定義對(duì)于`nn.Module`的forward方法的包裝 @functools.wraps(_orig_module_call) def module_call_wrapper(mod, *args, **kwargs): def forward(*args, **kwargs): return _orig_module_call(mod, *args, **kwargs) _autowrap_check( patcher, getattr(getattr(mod, "forward", mod), "__globals__", {}), self._autowrap_function_ids, ) return self.call_module(mod, forward, args, kwargs) # 這里Patcher的作用是在退出這一環(huán)境的時(shí)候恢復(fù)現(xiàn)場(chǎng),避免包裝函數(shù)、方法的影響溢出到`trace`之外。 with _Patcher() as patcher: # 對(duì)`__getattr__`和`nn.Module.__call__`這兩個(gè)方法默認(rèn)進(jìn)行包裝 patcher.patch_method( oneflow.nn.Module, "__getattr__", module_getattr_wrapper, deduplicate=False, ) patcher.patch_method( oneflow.nn.Module, "__call__", module_call_wrapper, deduplicate=False ) # 對(duì)預(yù)定好需要進(jìn)行包裝的函數(shù)進(jìn)行包裝 _patch_wrapped_functions(patcher) _autowrap_check(patcher, fn_globals, self._autowrap_function_ids) # 遍歷所有需要對(duì)其中函數(shù)進(jìn)行自動(dòng)包裝的package for module in self._autowrap_search: if module is oneflow: dict = {} # 當(dāng)package為oneflow時(shí),對(duì)此進(jìn)行特殊處理,單獨(dú)分出一個(gè)字典存放原本`oneflow.__dict__`中的內(nèi)容 for name, value in module.__dict__.items(): if not isinstance(value, oneflow.nn.Module) and not value in _oneflow_no_wrapped_functions: dict[name] = value _autowrap_check_oneflow( patcher, dict, module.__dict__, self._autowrap_function_ids ) else: _autowrap_check( patcher, module.__dict__, self._autowrap_function_ids ) # 創(chuàng)建節(jié)點(diǎn),這里的`create_node`調(diào)用實(shí)際上只是創(chuàng)建了最后一個(gè)節(jié)點(diǎn),即輸出節(jié)點(diǎn)。 # 但是這里`fn`就是forward函數(shù),在運(yùn)行這一函數(shù)的時(shí)候,就會(huì)如前面所說(shuō)依次創(chuàng)建節(jié)點(diǎn)。 self.create_node( "output", "output", (self.create_arg(fn(*args)),), {}, type_expr=fn.__annotations__.get("return", None), )?
其中,_patch_wrapped_functions的實(shí)現(xiàn)如下:
def _patch_wrapped_functions(patcher: _Patcher): # `_wrapped_fns_to_patch`中包含了所有需要自動(dòng)包裝的函數(shù) for frame_dict, name in _wrapped_fns_to_patch: if name not in frame_dict: if hasattr(builtins, name): # 對(duì)于built-in函數(shù),不存在于frame_dict中,單獨(dú)進(jìn)行處理來(lái)根據(jù)名稱獲取函數(shù)本身 orig_fn = getattr(builtins, name) else: # 如果是oneflow中指定需要包裝的函數(shù),那么就進(jìn)行獲取,否則拋出名稱無(wú)法識(shí)別的異常 is_oneflow_wrapped_function, func = is_oneflow_wrapped_function_and_try_get(name) if is_oneflow_wrapped_function: orig_fn = func else: raise NameError("Cannot deal with the function %s."%name) else: # 如果函數(shù)名稱已經(jīng)存在于frame_dict中,直接通過(guò)字典查詢來(lái)獲得函數(shù) orig_fn = frame_dict[name] # 創(chuàng)建包裝后的函數(shù)并進(jìn)行`patch`,即定義當(dāng)trace過(guò)程結(jié)束的時(shí)候,如何還原現(xiàn)場(chǎng) patcher.patch(frame_dict, name, _create_wrapped_func(orig_fn)) # 對(duì)于類中的方法,直接包裝并patch。 for cls, name in _wrapped_methods_to_patch: patcher.patch_method(cls, name, _create_wrapped_method(cls, name))
?
全局包裝
在模型的forward函數(shù)中,我們有時(shí)不僅會(huì)用到框架自帶的模塊或者函數(shù),有點(diǎn)時(shí)候還需要用到自定義的函數(shù)或者built-in函數(shù),對(duì)于這種情況如果不進(jìn)行處理,那么自然無(wú)法接受Proxy(x)的入?yún)ⅰx中提供了fx.wrap這一API,當(dāng)用戶需要調(diào)用這部分函數(shù)的時(shí)候,可以實(shí)現(xiàn)使用fx.wrap(func)使其被包裝。
例如:
import oneflow oneflow.fx.wrap(len) class MyModule(oneflow.nn.Module): def __init__(self): super().__init__() self.linear = oneflow.nn.Linear(512, 512) def forward(self, x): x = self.linear(x) + len(x.shape) return x traced = oneflow.fx.symbolic_trace(MyModule()) print(traced.code) """ def forward(self, x): linear = self.linear(x) getattr_1 = x.shape; x = None len_1 = len(getattr_1); getattr_1 = None add = linear + len_1; linear = len_1 = None return add """?
但是其局限性在于,如果Module的源代碼是來(lái)自其它庫(kù),那么在調(diào)用的地方使用fx.wrap是不起作用的,在oneflow和torch中都會(huì)有這一問(wèn)題。然而flowvision中有多處使用了built-in function,因此我們添加了一個(gè)API,即global_wrap,原理比較簡(jiǎn)單,就是直接對(duì)某個(gè)函數(shù)所在的包的__dict__進(jìn)行修改,用法如下:
# MyModule來(lái)自其它包 with oneflow.fx.global_wrap(len): m = MyModule() traced = oneflow.fx.symbolic_trace(m) print(traced.code) """ def forward(self, x): linear = self.linear(x); x = None getattr_1 = linear.shape len_1 = len(getattr_1); getattr_1 = None relu = oneflow.relu(linear); linear = None add = relu + len_1; relu = len_1 = None return add """?
使用with關(guān)鍵字的原因是這種實(shí)現(xiàn)方式是直接修改了某個(gè)包的__dict__,對(duì)于其它地方的調(diào)用也會(huì)產(chǎn)生影響,因此需要將其限制在一定范圍內(nèi)。此外,包裝后的函數(shù)包含了對(duì)類型的判定等一系列操作,也會(huì)極大影響built-in函數(shù)的性能。
其它適配
其它地方的處理都比較簡(jiǎn)單,不需要對(duì)實(shí)現(xiàn)方式做修改,只需要將細(xì)節(jié)部分對(duì)齊即可,這也體現(xiàn)出oneflow和pytorch在前端部分的高度兼容性。
4
IR設(shè)計(jì)
fx的IR設(shè)計(jì)遵循以下幾個(gè)原則:
避免支持長(zhǎng)尾分布,復(fù)雜的樣例。主要關(guān)注經(jīng)典模型的程序捕獲和變換。
使用機(jī)器學(xué)習(xí)從業(yè)者已經(jīng)熟悉的工具和概念,例如Python的數(shù)據(jù)結(jié)構(gòu)和 PyTorch 中公開記錄的算子 。
使程序捕獲過(guò)程具有高度可配置性,以便用戶可以為長(zhǎng)尾需求實(shí)現(xiàn)自己的解決方案。
fx的IR主要由幾個(gè)部分組成;
opcode:即當(dāng)前操作的類型,可以是placeholder, get_attr, call_function, call_method, call_module, output
name:即給當(dāng)前操作的命名。
target:當(dāng)前操作的實(shí)體,例如對(duì)于call_function類型的操作,可能這一屬性會(huì)是
args和kwargs:指定當(dāng)前操作的參數(shù)。
通過(guò)print_tabular這一API可以很方便美觀地打印出fx中的IR,例如對(duì)于以下的MyModule模型,我們可以打印出其IR:
import oneflow class MyModule(oneflow.nn.Module): def __init__(self, do_activation : bool = False): super().__init__() self.do_activation = do_activation self.linear = oneflow.nn.Linear(512, 512) def forward(self, x): x = self.linear(x) y = oneflow.ones([2, 3]) x = oneflow.topk(x, 10) return x.relu() + y traced = oneflow.fx.symbolic_trace(MyModule()) traced.graph.print_tabular() """ opcode name target args kwargs ------------- ----------------- ------------------------ ------------------------- -------- placeholder x x () {} call_module linear linear (x,) {} call_function topk(linear, 10) {} call_method relu relu (topk,) {} get_attr _tensor_constant0 _tensor_constant0 () {} call_function add (relu, _tensor_constant0) {} output output output (add,) {} """
盡管fx的IR不算強(qiáng)大(例如不能處理動(dòng)態(tài)控制流),但是定義非常簡(jiǎn)潔,實(shí)現(xiàn)簡(jiǎn)單,對(duì)于用戶來(lái)講上手門檻相對(duì)低很多。
5
One-fx應(yīng)用舉例
OP替換
下面的例子展示了如何將add操作全部替換成mul操作。
import oneflow from oneflow.fx import symbolic_trace import operator class M(oneflow.nn.Module): def forward(self, x, y): return x + y, oneflow.add(x, y), x.add(y) if __name__ == '__main__': traced = symbolic_trace(M()) patterns = set([operator.add, oneflow.add, "add"]) for n in traced.graph.nodes: if any(n.target == pattern for pattern in patterns): with traced.graph.inserting_after(n): new_node = traced.graph.call_function(oneflow.mul, n.args, n.kwargs) n.replace_all_uses_with(new_node) traced.graph.erase_node(n) traced.recompile() traced.graph.print_tabular() print(traced.code)
?
性能分析
以下代碼展示如何使用fx進(jìn)行模型的性能分析,將原本的模型通過(guò)symbolic_trace解析成各個(gè)節(jié)點(diǎn),再在其中插入測(cè)試性能的操作。
import oneflow import flowvision.models as models import statistics, tabulate, time from typing import Any, Dict, List class ProfilingInterpreter(oneflow.fx.Interpreter): def __init__(self, mod : oneflow.nn.Module): gm = oneflow.fx.symbolic_trace(mod) super().__init__(gm) # 記錄總運(yùn)行時(shí)間 self.total_runtime_sec : List[float] = [] # 記錄各個(gè)節(jié)點(diǎn)運(yùn)行時(shí)間 self.runtimes_sec : Dict[oneflow.fx.Node, List[float]] = {} # 重寫`run`方法,本質(zhì)上是對(duì)基類`run`方法的簡(jiǎn)單封裝,在運(yùn)行前后記錄時(shí)間點(diǎn)。 # 這一方法是Graph整體運(yùn)行的入口。 def run(self, *args) -> Any: t_start = time.time() return_val = super().run(*args) t_end = time.time() self.total_runtime_sec.append(t_end - t_start) return return_val # 同上,重寫`run_node`方法,不需要自己寫細(xì)節(jié)實(shí)現(xiàn),只需要在對(duì)基類的`run_node`調(diào)用前后記錄時(shí)間點(diǎn)即可 # 這一方法是Graph中運(yùn)行每個(gè)Node的入口。 def run_node(self, n : oneflow.fx.Node) -> Any: t_start = time.time() return_val = super().run_node(n) t_end = time.time() self.runtimes_sec.setdefault(n, []) self.runtimes_sec[n].append(t_end - t_start) return return_val # 定義如何打印性能測(cè)試結(jié)果 def summary(self, should_sort : bool = False) -> str: # 存儲(chǔ)每個(gè)節(jié)點(diǎn)的打印信息 node_summaries : List[List[Any]] = [] # 由于模塊會(huì)被調(diào)用多次,所以這里計(jì)算一下平均的運(yùn)行總時(shí)長(zhǎng) mean_total_runtime = statistics.mean(self.total_runtime_sec) for node, runtimes in self.runtimes_sec.items(): mean_runtime = statistics.mean(runtimes) # 計(jì)算節(jié)點(diǎn)運(yùn)行時(shí)間占總時(shí)間的比例 pct_total = mean_runtime / mean_total_runtime * 100 # 記錄節(jié)點(diǎn)信息、節(jié)點(diǎn)平均運(yùn)行時(shí)長(zhǎng)和節(jié)點(diǎn)運(yùn)行時(shí)間占總時(shí)間的比例 node_summaries.append( [node.op, str(node), mean_runtime, pct_total]) # 如果需要,安按照運(yùn)行時(shí)間進(jìn)行排序 if should_sort: node_summaries.sort(key=lambda s: s[2], reverse=True) # 以下是借助tabulate庫(kù)進(jìn)行格式化來(lái)美化顯示效果 headers : List[str] = [ 'Op type', 'Op', 'Average runtime (s)', 'Pct total runtime' ] return tabulate.tabulate(node_summaries, headers=headers) if __name__ == '__main__': rn18 = models.resnet18() rn18.eval() input = oneflow.randn(5, 3, 224, 224) output = rn18(input) interp = ProfilingInterpreter(rn18) interp.run(input) print(interp.summary(True))
?
效果如下:
算子融合
以下代碼演示如何借助fx將模型中的卷積層和BN層進(jìn)行融合,對(duì)于這種組合,并不需要引入新的算子,只需要對(duì)原本conv的權(quán)重進(jìn)行操作即可。
import sys import oneflow import oneflow.nn as nn import numpy as np import copy from typing import Dict, Any, Tuple # 通過(guò)直接對(duì)權(quán)重進(jìn)行運(yùn)算的方式進(jìn)行Conv和BN的融合 def fuse_conv_bn_eval(conv, bn): assert(not (conv.training or bn.training)), "Fusion only for eval!" fused_conv = copy.deepcopy(conv) fused_conv.weight, fused_conv.bias = fuse_conv_bn_weights(fused_conv.weight, fused_conv.bias, bn.running_mean, bn.running_var, bn.eps, bn.weight, bn.bias) return fused_conv # 權(quán)重融合方式 def fuse_conv_bn_weights(conv_w, conv_b, bn_rm, bn_rv, bn_eps, bn_w, bn_b): if conv_b is None: conv_b = oneflow.zeros_like(bn_rm) if bn_w is None: bn_w = oneflow.ones_like(bn_rm) if bn_b is None: bn_b = oneflow.zeros_like(bn_rm) bn_var_rsqrt = oneflow.rsqrt(bn_rv + bn_eps) conv_w = conv_w * (bn_w * bn_var_rsqrt).reshape([-1] + [1] * (len(conv_w.shape) - 1)) conv_b = (conv_b - bn_rm) * bn_var_rsqrt * bn_w + bn_b return oneflow.nn.Parameter(conv_w), oneflow.nn.Parameter(conv_b) # 根據(jù)字符串對(duì)名稱進(jìn)行分割,比如`foo.bar.baz` -> (`foo.bar`, `baz`) def _parent_name(target : str) -> Tuple[str, str]: *parent, name = target.rsplit('.', 1) return parent[0] if parent else '', name def replace_node_module(node: oneflow.fx.Node, modules: Dict[str, Any], new_module: oneflow.nn.Module): assert(isinstance(node.target, str)) parent_name, name = _parent_name(node.target) setattr(modules[parent_name], name, new_module) # 定義對(duì)模型進(jìn)行融合操作的過(guò)程 def fuse(model: oneflow.nn.Module) -> oneflow.nn.Module: model = copy.deepcopy(model) # 先通過(guò)fx.symbolic_trace獲取一個(gè)GraphModule fx_model: oneflow.fx.GraphModule = oneflow.fx.symbolic_trace(model) modules = dict(fx_model.named_modules()) # 遍歷GraphModule中的所有節(jié)點(diǎn),分別進(jìn)行操作 for node in fx_model.graph.nodes: # 跳過(guò)所有不是module的節(jié)點(diǎn) if node.op != 'call_module': continue # 檢測(cè)到conv+bn的結(jié)構(gòu)后進(jìn)行融合操作 if type(modules[node.target]) is nn.BatchNorm2d and type(modules[node.args[0].target]) is nn.Conv2d: # conv的輸出同時(shí)被其它節(jié)點(diǎn)使用,即conv后連接兩個(gè)節(jié)點(diǎn)時(shí)無(wú)法融合 if len(node.args[0].users) > 1: continue conv = modules[node.args[0].target] bn = modules[node.target] fused_conv = fuse_conv_bn_eval(conv, bn) replace_node_module(node.args[0], modules, fused_conv) # 對(duì)圖中的邊進(jìn)行置換,對(duì)于用到bn輸出的節(jié)點(diǎn),要更改它們的輸入 node.replace_all_uses_with(node.args[0]) # 移除舊的節(jié)點(diǎn) fx_model.graph.erase_node(node) fx_model.graph.lint() # 重新建圖(構(gòu)造模型) fx_model.recompile() return fx_model if __name__ == '__main__': # 以下引入flowvision中的resnet 18模型,并進(jìn)行融合前后的benchmark比較 import flowvision.models as models import time rn18 = models.resnet18().cuda() rn18.eval() inp = oneflow.randn(10, 3, 224, 224).cuda() output = rn18(inp) def benchmark(model, iters=20): for _ in range(10): model(inp) oneflow.cuda.synchronize() begin = time.time() for _ in range(iters): model(inp) return str(time.time()-begin) fused_rn18 = fuse(rn18) unfused_time = benchmark(rn18) fused_time = benchmark(fused_rn18) print("Unfused time: ", benchmark(rn18)) print("Fused time: ", benchmark(fused_rn18)) assert unfused_time > fused_time?
6
未來(lái)計(jì)劃
基于fx進(jìn)行8bit量化感知訓(xùn)練和部署
基于fx進(jìn)行算子融合
eager模式下基于fx獲得模型更精確的FLOPs和MACs結(jié)果
審核編輯:劉清
-
python
+關(guān)注
關(guān)注
56文章
4807瀏覽量
84961 -
pytorch
+關(guān)注
關(guān)注
2文章
808瀏覽量
13331 -
OneFlow
+關(guān)注
關(guān)注
0文章
9瀏覽量
8806
原文標(biāo)題:適配PyTorch FX,OneFlow讓量化感知訓(xùn)練更簡(jiǎn)單
文章出處:【微信號(hào):GiantPandaCV,微信公眾號(hào):GiantPandaCV】歡迎添加關(guān)注!文章轉(zhuǎn)載請(qǐng)注明出處。
發(fā)布評(píng)論請(qǐng)先 登錄
相關(guān)推薦
Pytorch模型訓(xùn)練實(shí)用PDF教程【中文】
YOLOv6中的用Channel-wise Distillation進(jìn)行的量化感知訓(xùn)練
9個(gè)用Pytorch訓(xùn)練快速神經(jīng)網(wǎng)絡(luò)的技巧
如何讓PyTorch模型訓(xùn)練變得飛快?
Pytorch量化感知訓(xùn)練的詳解
基于PyTorch的深度學(xué)習(xí)入門教程之PyTorch簡(jiǎn)單知識(shí)
PyTorch教程之15.2近似訓(xùn)練
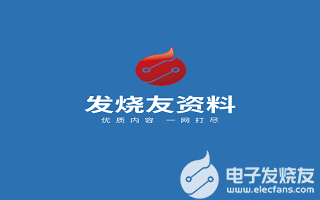
PyTorch教程15.10之預(yù)訓(xùn)練BERT
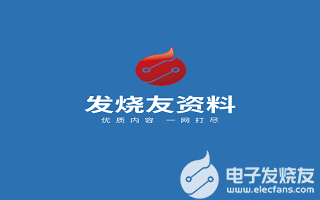
PyTorch教程21.7之序列感知推薦系統(tǒng)
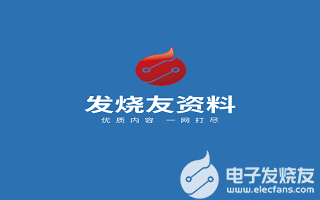
評(píng)論