1.導(dǎo)入需要的包和基本配置
importargparse#解析命令行參數(shù)模塊 importjson#字典列表和JSON字符串之間的相互解析模塊 importos#與操作系統(tǒng)進(jìn)行交互的模塊包含文件路徑操作和解析 importsys#sys系統(tǒng)模塊包含了與Python解釋器和它的環(huán)境有關(guān)的函數(shù) frompathlibimportPath#Path將str轉(zhuǎn)換為Path對(duì)象使字符串路徑易于操作的模塊 importnumpyasnp#NumPy(NumericalPython)是Python的一種開源的數(shù)值計(jì)算擴(kuò)展 importoneflowasflow#OneFlow深度學(xué)習(xí)框架 fromtqdmimporttqdm#進(jìn)度條模塊 frommodels.commonimportDetectMultiBackend#下面都是one-yolov5定義的模塊,在本系列的其它文章都有涉及 fromutils.callbacksimportCallbacks fromutils.dataloadersimportcreate_dataloader fromutils.generalimport( LOGGER, check_dataset, check_img_size, check_requirements, check_yaml, coco80_to_coco91_class, colorstr, increment_path, non_max_suppression, print_args, scale_coords, xywh2xyxy, xyxy2xywh, ) fromutils.metricsimportConfusionMatrix,ap_per_class,box_iou fromutils.oneflow_utilsimportselect_device,time_sync fromutils.plotsimportoutput_to_target,plot_images,plot_val_study
2.opt參數(shù)詳解
參數(shù) | 解析 | |
---|---|---|
data | dataset.yaml path | 數(shù)據(jù)集配置文件地址 包含數(shù)據(jù)集的路徑、類別個(gè)數(shù)、類名、下載地址等信息 |
weights | model weights path(s) | 模型的權(quán)重文件地址 weights/yolov5s |
batch-size | batch size | 計(jì)算樣本的批次大小 默認(rèn)32 |
imgsz | inference size (pixels) | 輸入網(wǎng)絡(luò)的圖片分辨率 默認(rèn)640 |
conf-thres | confidence threshold | object置信度閾值 默認(rèn)0.001 |
iou-thres | NMS IoU threshold | 進(jìn)行NMS時(shí)IOU的閾值 默認(rèn)0.6 |
task | train, val, test, speed or study | 設(shè)置測(cè)試的類型 有train, val, test, speed or study幾種 默認(rèn)val |
device | cuda device, i.e. 0 or 0,1,2,3 or cpu | 測(cè)試的設(shè)備 |
workers | max dataloader workers (per RANK in DDP mode) | 加載數(shù)據(jù)使用的 dataloader workers |
single-cls | treat as single-class dataset | 數(shù)據(jù)集是否只用一個(gè)類別 默認(rèn)False |
augment | augmented inference | 測(cè)試是否使用TTA Test Time Augment 默認(rèn)False |
verbose | report mAP by class | 是否打印出每個(gè)類別的mAP 默認(rèn)False |
save-hybrid | save label+prediction hybrid results to *.txt | 保存label+prediction 雜交結(jié)果到對(duì)應(yīng).txt 默認(rèn)False |
save-conf | save confidences in --save-txt labels | |
save-json | save a COCO-JSON results file | 是否按照coco的json格式保存結(jié)果 默認(rèn)False |
project | save to project/name | 測(cè)試保存的源文件 默認(rèn)runs/val |
name | save to project/name | 測(cè)試保存的文件地址名 默認(rèn)exp 保存在runs/val/exp下 |
exist-ok | existing project/name ok, do not increment | 是否保存在當(dāng)前文件,不新增 默認(rèn)False |
half | use FP16 half-precision inference | 是否使用半精度推理 默認(rèn)False |
dnn | use OpenCV DNN for ONNX inference | 是否使用 OpenCV DNN 對(duì) ONNX 模型推理 |
3.main函數(shù)
根據(jù)解析的opt參數(shù),調(diào)用run函數(shù)
defmain(opt): #檢測(cè)requirements文件中需要的包是否安裝好了 check_requirements(requirements=ROOT/"requirements.txt",exclude=("tensorboard","thop")) ifopt.taskin("train","val","test"):#runnormally ifopt.conf_thres>0.001:#更多請(qǐng)見https://github.com/ultralytics/yolov5/issues/1466 LOGGER.info(f"WARNING:confidencethreshold{opt.conf_thres}>0.001producesinvalidresults") run(**vars(opt)) else: weights=opt.weightsifisinstance(opt.weights,list)else[opt.weights] opt.half=True#FP16forfastestresults ifopt.task=="speed":#speedbenchmarks #pythonval.py--taskspeed--datacoco.yaml #--batch1--weightsyolov5n/yolov5s/... opt.conf_thres,opt.iou_thres,opt.save_json=0.25,0.45,False foropt.weightsinweights: run(**vars(opt),plots=False) elifopt.task=="study":#speedvsmAPbenchmarks #pythonval.py--taskstudy--datacoco.yaml #--iou0.7--weightsyolov5n/yolov5s/... foropt.weightsinweights: f=f"study_{Path(opt.data).stem}_{Path(opt.weights).stem}.txt" x,y=( list(range(256,1536+128,128)), [], )#xaxis(imagesizes),yaxis #"study":模型在各個(gè)尺度下的指標(biāo)并可視化, #上面list(range(256,1536+128,128)),代表img-size的各個(gè)尺度,具體代碼如下: foropt.imgszinx:#img-size LOGGER.info(f" Running{f}--imgsz{opt.imgsz}...") r,_,t=run(**vars(opt),plots=False) y.append(r+t)#resultsandtimes np.savetxt(f,y,fmt="%10.4g")#save os.system("zip-rstudy.zipstudy_*.txt") #可視化各個(gè)指標(biāo) plot_val_study(x=x)#plot
3. run函數(shù)
3.1 載入?yún)?shù)
#不參與反向傳播 @flow.no_grad() defrun( data,#數(shù)據(jù)集配置文件地址包含數(shù)據(jù)集的路徑、類別個(gè)數(shù)、類名、下載地址等信息train.py時(shí)傳入data_dict weights=None,#模型的權(quán)重文件地址運(yùn)行train.py=None運(yùn)行test.py=默認(rèn)weights/yolov5s batch_size=32,#前向傳播的批次大小運(yùn)行test.py傳入默認(rèn)32運(yùn)行train.py則傳入batch_size//WORLD_SIZE*2 imgsz=640,#輸入網(wǎng)絡(luò)的圖片分辨率運(yùn)行test.py傳入默認(rèn)640運(yùn)行train.py則傳入imgsz_test conf_thres=0.001,#object置信度閾值默認(rèn)0.001 iou_thres=0.6,#進(jìn)行NMS時(shí)IOU的閾值默認(rèn)0.6 task="val",#設(shè)置測(cè)試的類型有train,val,test,speedorstudy幾種默認(rèn)val device="",#執(zhí)行val.py所在的設(shè)備cudadevice,i.e.0or0,1,2,3orcpu workers=8,#dataloader中的最大worker數(shù)(線程個(gè)數(shù)) single_cls=False,#數(shù)據(jù)集是否只有一個(gè)類別默認(rèn)False augment=False,#測(cè)試時(shí)增強(qiáng),詳細(xì)請(qǐng)看我們的教程:https://start.oneflow.org/oneflow-yolo-doc/tutorials/03_chapter/TTA.html verbose=False,#是否打印出每個(gè)類別的mAP運(yùn)行test.py傳入默認(rèn)Fasle運(yùn)行train.py則傳入nc50?and?final_epoch ????save_txt=False,??#?是否以txt文件的形式保存模型預(yù)測(cè)框的坐標(biāo)?默認(rèn)True ????save_hybrid=False,??#?是否save?label+prediction?hybrid?results?to?*.txt??默認(rèn)False ????save_conf=False,??#?是否保存預(yù)測(cè)每個(gè)目標(biāo)的置信度到預(yù)測(cè)txt文件中?默認(rèn)True ????save_json=False,??#?是否按照coco的json格式保存預(yù)測(cè)框,并且使用cocoapi做評(píng)估(需要同樣coco的json格式的標(biāo)簽), ??????????????????????#運(yùn)行test.py傳入默認(rèn)Fasle?運(yùn)行train.py則傳入is_coco?and?final_epoch(一般也是False) ????project=ROOT?/?"runs/val",??#?驗(yàn)證結(jié)果保存的根目錄?默認(rèn)是?runs/val ????name="exp",???#?驗(yàn)證結(jié)果保存的目錄?默認(rèn)是exp??最終:?runs/val/exp ????exist_ok=False,??#?如果文件存在就increment?name,不存在就新建??默認(rèn)False(默認(rèn)文件都是不存在的) ????half=True,????#?使用?FP16?的半精度推理 ????dnn=False,????#?在?ONNX?推理時(shí)使用?OpenCV?DNN?后段端 ????model=None,???#?如果執(zhí)行val.py就為None?如果執(zhí)行train.py就會(huì)傳入(?model=attempt_load(f,?device).half()?) ????dataloader=None,???#?數(shù)據(jù)加載器?如果執(zhí)行val.py就為None?如果執(zhí)行train.py就會(huì)傳入testloader ????save_dir=Path(""),?#?文件保存路徑?如果執(zhí)行val.py就為‘’?,?如果執(zhí)行train.py就會(huì)傳入save_dir(runs/train/expn) ????plots=True,??#?是否可視化?運(yùn)行val.py傳入,默認(rèn)True? ????callbacks=Callbacks(),? ????compute_loss=None,?#?損失函數(shù)?運(yùn)行val.py傳入默認(rèn)None?運(yùn)行train.py則傳入compute_loss(train) ):
3.2 Initialize/load model and set device(初始化/加載模型以及設(shè)置設(shè)備)
iftraining:#通過train.py調(diào)用的run函數(shù) device,of,engine=( next(model.parameters()).device, True, False, )#getmodeldevice,OneFlowmodel half&=device.type!="cpu"#halfprecisiononlysupportedonCUDA model.half()ifhalfelsemodel.float() else:#直接通過val.py調(diào)用run函數(shù) device=select_device(device,batch_size=batch_size) #Directories生成save_dir文件路徑run/val/expn save_dir=increment_path(Path(project)/name,exist_ok=exist_ok)#incrementrun (save_dir/"labels"ifsave_txtelsesave_dir).mkdir(parents=True,exist_ok=True)#makedir #加載模型只在運(yùn)行val.py才需要自己加載model model=DetectMultiBackend(weights,device=device,dnn=dnn,data=data,fp16=half) stride,of,engine=model.stride,model.of,model.engine #檢測(cè)輸入圖片的分辨率imgsz是否能被stride整除 imgsz=check_img_size(imgsz,s=stride)#checkimagesize half=model.fp16#FP16supportedonlimitedbackendswithCUDA ifengine: batch_size=model.batch_size else: device=model.device ifnotof: batch_size=1#export.pymodelsdefaulttobatch-size1 LOGGER.info(f"Forcing--batch-size1inference(1,3,{imgsz},{imgsz})fornon-OneFlowmodels") #Data data=check_dataset(data)#check
3.3 Configure
#配置 model.eval()#啟動(dòng)模型驗(yàn)證模式 cuda=device.type!="cpu" is_coco=isinstance(data.get("val"),str)anddata["val"].endswith(f"coco{os.sep}val2017.txt")#通過COCO數(shù)據(jù)集的文件夾組織結(jié)構(gòu)判斷當(dāng)前數(shù)據(jù)集是否為COCO數(shù)據(jù)集 nc=1ifsingle_clselseint(data["nc"])#numberofclasses #設(shè)置iou閾值從0.5-0.95取10個(gè)(0.05間隔)iouvectorformAP@0.5:0.95 #iouv:[0.50000,0.55000,0.60000,0.65000,0.70000,0.75000,0.80000,0.85000,0.90000,0.95000] iouv=flow.linspace(0.5,0.95,10,device=device)#iouvectorformAP@0.5:0.95 niou=iouv.numel()#示例mAP@0.5:0.95iou閾值個(gè)數(shù)=10個(gè),計(jì)算mAP的詳細(xì)教程可以在https://start.oneflow.org/oneflow-yolo-doc/tutorials/05_chapter/map_analysis.html這里查看
3.4 Dataloader
通過 train.py 調(diào)用 run 函數(shù)會(huì)傳入一個(gè) Dataloader,而通過 val.py 需要加載測(cè)試數(shù)據(jù)集
#Dataloader #如果不是訓(xùn)練(執(zhí)行val.py腳本調(diào)用run函數(shù))就調(diào)用create_dataloader生成dataloader #如果是訓(xùn)練(執(zhí)行train.py調(diào)用run函數(shù))就不需要生成dataloader可以直接從參數(shù)中傳過來testloader ifnottraining:#加載val數(shù)據(jù)集 ifofandnotsingle_cls:#check--weightsaretrainedon--data ncm=model.model.nc assertncm==nc,( f"{weights}({ncm}classes)trainedondifferent--datathanwhatyoupassed({nc}"f"classes).Passcorrectcombinationof"f"--weightsand--datathataretrainedtogether." ) model.warmup(imgsz=(1ifofelsebatch_size,3,imgsz,imgsz))#warmup pad=0.0iftaskin("speed","benchmark")else0.5 rect=Falseiftask=="benchmark"elseof#squareinferenceforbenchmarks task=taskiftaskin("train","val","test")else"val"#pathtotrain/val/testimages #創(chuàng)建dataloader這里的rect默認(rèn)為True矩形推理用于測(cè)試集在不影響mAP的情況下可以大大提升推理速度 dataloader=create_dataloader( data[task], imgsz, batch_size, stride, single_cls, pad=pad, rect=rect, workers=workers, prefix=colorstr(f"{task}:"), )[0]
3.5 初始化
#初始化驗(yàn)證的圖片的數(shù)量 seen=0 #初始化混淆矩陣 confusion_matrix=ConfusionMatrix(nc=nc) #獲取數(shù)據(jù)集所有目標(biāo)類別的類名 names=dict(enumerate(model.namesifhasattr(model,"names")elsemodel.module.names)) #coco80_to_coco91_class:converts80-index(val2014)to91-index(paper) #https://tech.amikelive.com/node-718/what-object-categories-labels-are-in-coco-dataset/ class_map=coco80_to_coco91_class()ifis_cocoelselist(range(1000)) #設(shè)置進(jìn)度條模塊顯示信息 s=("%20s"+"%11s"*6)%( "Class", "Images", "Labels", "P", "R", "mAP@.5", "mAP@.5:.95", ) #初始化時(shí)間dt[t0(預(yù)處理的時(shí)間),t1(推理的時(shí)間),t2(后處理的時(shí)間)]和p,r,f1,mp,mr,map50,map指標(biāo) dt,p,r,f1,mp,mr,map50,map=( [0.0,0.0,0.0], 0.0, 0.0, 0.0, 0.0, 0.0, 0.0, 0.0, ) #初始化驗(yàn)證集的損失 loss=flow.zeros(3,device=device) #初始化json文件中的字典,統(tǒng)計(jì)信息,ap,ap_class jdict,stats,ap,ap_class=[],[],[],[] callbacks.run("on_val_start") #初始化tqdm進(jìn)度條模塊 pbar=tqdm(dataloader,desc=s,bar_format="{l_bar}{bar:10}{r_bar}{bar:-10b}")示例輸出
val:data=data/coco.yaml,weights=['yolov5x'],batch_size=32,imgsz=640,conf_thres=0.001,iou_thres=0.6,task=val, device=,workers=8,single_cls=False,augment=False,verbose=False,save_txt=False,save_hybrid=False, save_conf=False,save_json=True,project=runs/val,name=exp,exist_ok=False,half=True,dnn=False YOLOv5v1.0-8-g94ec5c4Python-3.8.13oneflow-0.8.1.dev20221018+cu112 Fusinglayers... Modelsummary:322layers,86705005parameters,571965gradients val:Scanning'/data/dataset/fengwen/coco/val2017.cache'imagesandlabels...4952found,48missing,0empty,0corrupt:100%|████████ ClassImagesLabelsPRmAP@.5mAP@.5:.95:100%|██████████|157/157[01:55<00:00,??1.36it/ ?????????????????all???????5000??????36335??????0.743??????0.627??????0.685??????0.503 Speed:?0.1ms?pre-process,?7.5ms?inference,?2.1ms?NMS?per?image?at?shape?(32,?3,?640,?640)??#?<---?baseline?speed Evaluating?pycocotools?mAP...?saving?runs/val/exp3/yolov5x_predictions.json... ... Average?Precision??(AP)?@[?IoU=0.50:0.95?|?area=???all?|?maxDets=100?]?=?0.505?#?<---?baseline?mAP ?Average?Precision??(AP)?@[?IoU=0.50??????|?area=???all?|?maxDets=100?]?=?0.689 ?Average?Precision??(AP)?@[?IoU=0.75??????|?area=???all?|?maxDets=100?]?=?0.545 ?Average?Precision??(AP)?@[?IoU=0.50:0.95?|?area=?small?|?maxDets=100?]?=?0.339 ?Average?Precision??(AP)?@[?IoU=0.50:0.95?|?area=medium?|?maxDets=100?]?=?0.557 ?Average?Precision??(AP)?@[?IoU=0.50:0.95?|?area=?large?|?maxDets=100?]?=?0.650 ?Average?Recall?????(AR)?@[?IoU=0.50:0.95?|?area=???all?|?maxDets=??1?]?=?0.382 ?Average?Recall?????(AR)?@[?IoU=0.50:0.95?|?area=???all?|?maxDets=?10?]?=?0.628 ?Average?Recall?????(AR)?@[?IoU=0.50:0.95?|?area=???all?|?maxDets=100?]?=?0.677??#?<---?baseline?mAR ?Average?Recall?????(AR)?@[?IoU=0.50:0.95?|?area=?small?|?maxDets=100?]?=?0.523 ?Average?Recall?????(AR)?@[?IoU=0.50:0.95?|?area=medium?|?maxDets=100?]?=?0.730 ?Average?Recall?????(AR)?@[?IoU=0.50:0.95?|?area=?large?|?maxDets=100?]?=?0.826
3.6 開始驗(yàn)證
forbatch_i,(im,targets,paths,shapes)inenumerate(pbar): """https://github.com/Oneflow-Inc/one-yolov5/blob/bf8c66e011fcf5b8885068074ffc6b56c113a20c/utils/dataloaders.py#L735 im:flow.from_numpy(img); targets:labels_out paths:self.im_files[index] shapes:shapes """
3.6.1 驗(yàn)證開始前的預(yù)處理
callbacks.run("on_val_batch_start") t1=time_sync() ifcuda: im=im.to(device) targets=targets.to(device) im=im.half()ifhalfelseim.float()#uint8tofp16/32 im/=255#0-255to0.0-1.0 nb,_,height,width=im.shape#batchsize,channels,height,width t2=time_sync() dt[0]+=t2-t1
3.6.2 推理
#Inference out,train_out=model(im)iftrainingelsemodel(im,augment=augment,val=True)#輸出為:推理結(jié)果、損失值 dt[1]+=time_sync()-t2
3.6.3 計(jì)算損失
#Loss """ 分類損失(cls_loss):該損失用于判斷模型是否能夠準(zhǔn)確地識(shí)別出圖像中的對(duì)象,并將其分類到正確的類別中。 置信度損失(obj_loss):該損失用于衡量模型預(yù)測(cè)的框(即包含對(duì)象的矩形)與真實(shí)框之間的差異。 邊界框損失(box_loss):該損失用于衡量模型預(yù)測(cè)的邊界框與真實(shí)邊界框之間的差異,這有助于確保模型能夠準(zhǔn)確地定位對(duì)象。 """ ifcompute_loss: loss+=compute_loss([x.float()forxintrain_out],targets)[1]#box,obj,cls
3.6.4 Run NMS
#NMS #將真實(shí)框target的xywh(因?yàn)閠arget是在labelimg中做了歸一化的)映射到真實(shí)的圖像(test)尺寸 targets[:,2:]*=flow.tensor((width,height,width,height),device=device)#topixels #在NMS之前將數(shù)據(jù)集標(biāo)簽targets添加到模型預(yù)測(cè)中,這允許在數(shù)據(jù)集中自動(dòng)標(biāo)記(forautolabelling)其它對(duì)象(在pred中混入gt)并且mAP反映了新的混合標(biāo)簽 #targets:[num_target,img_index+class_index+xywh]=[31,6] #lb:{list:bs}第一張圖片的target[17,5]第二張[1,5]第三張[7,5]第四張[6,5] lb=[targets[targets[:,0]==i,1:]foriinrange(nb)]ifsave_hybridelse[]#forautolabelling t3=time_sync() """non_max_suppression(非最大值抑制) Non-MaximumSuppression(NMS)oninferenceresultstorejectoverlappingboundingboxes 該算法的原理: 先假設(shè)有6個(gè)矩形框,根據(jù)分類器的類別分類概率大小排序,假設(shè)從小到大屬于車輛(被檢測(cè)的目標(biāo))的概率分別為:A、B、C、D、E、F (1)從最大概率矩形框F開始,分別判斷A~E與F的重疊度IOU是否大于某個(gè)指定的閥值; (2)假設(shè)B、D與F的重疊度大于指定的閥值,則丟棄B、D,并標(biāo)記第一個(gè)矩形框F,是我們要保留的 (3)從剩下的矩形框A、C、E中,選擇最大概率,假設(shè)為E,然后判斷A、C與E的重疊度是否大于指定的閥值, 假如大于就丟棄A、C,并標(biāo)記E,是我們保留下來的第二個(gè)矩形框 一直重復(fù)上述過程,找到所有被保留的矩形框 Returns: listofdetections,on(n,6)tensorperimage[xyxy,conf,cls] """ out=non_max_suppression(out,conf_thres,iou_thres,labels=lb,multi_label=True,agnostic=single_cls) #獲取NMS時(shí)間 dt[2]+=time_sync()-t3
3.6.5 統(tǒng)計(jì)每張圖片的真實(shí)框、預(yù)測(cè)框信息
#為每張圖片做統(tǒng)計(jì),寫入預(yù)測(cè)信息到txt文件,生成json文件字典,統(tǒng)計(jì)tp等 #out:list{bs}[300,6][42,6][300,6][300,6][:,image_index+class+xywh] forsi,predinenumerate(out): #獲取第si張圖片的gt標(biāo)簽信息包括class,x,y,w,htarget[:,0]為標(biāo)簽屬于哪張圖片的編號(hào) labels=targets[targets[:,0]==si,1:]#[:,class+xywh] nl,npr=labels.shape[0],pred.shape[0]#numberoflabels,predictions path,shape=Path(paths[si]),shapes[si][0] correct=flow.zeros(npr,niou,dtype=flow.bool,device=device)#init seen+=1#統(tǒng)計(jì)測(cè)試圖片數(shù)量+1 ifnpr==0:#如果預(yù)測(cè)為空,則添加空的信息到stats里 ifnl: stats.append((correct,*flow.zeros((2,0),device=device),labels[:,0])) ifplots: confusion_matrix.process_batch(detections=None,labels=labels[:,0]) continue #Predictions ifsingle_cls: pred[:,5]=0 predn=pred.clone() #將預(yù)測(cè)坐標(biāo)映射到原圖img中 scale_coords(im[si].shape[1:],predn[:,:4],shape,shapes[si][1])#native-spacepred #Evaluate ifnl: tbox=xywh2xyxy(labels[:,1:5])#targetboxes scale_coords(im[si].shape[1:],tbox,shape,shapes[si][1])#native-spacelabels labelsn=flow.cat((labels[:,0:1],tbox),1)#native-spacelabels correct=process_batch(predn,labelsn,iouv) ifplots: confusion_matrix.process_batch(predn,labelsn) stats.append((correct,pred[:,4],pred[:,5],labels[:,0]))#(correct,conf,pcls,tcls) #Save/log #保存預(yù)測(cè)信息到txt文件runsvalexp7labelsimage_name.txt ifsave_txt: save_one_txt( predn, save_conf, shape, file=save_dir/"labels"/f"{path.stem}.txt", ) ifsave_json: save_one_json(predn,jdict,path,class_map)#appendtoCOCO-JSONdictionary callbacks.run("on_val_image_end",pred,predn,path,names,im[si])
3.6.6 畫出前三個(gè)batch圖片的 gt 和 pred 框
gt : 真實(shí)框,Ground truth box, 是人工標(biāo)注的位置,存放在標(biāo)注文件中
pred : 預(yù)測(cè)框,Prediction box, 是由目標(biāo)檢測(cè)模型計(jì)算輸出的框
#Plotimages ifplotsandbatch_i3: ????plot_images(im,?targets,?paths,?save_dir?/?f"val_batch{batch_i}_labels.jpg",?names)??#?labels ????plot_images( ????????im, ????????output_to_target(out), ????????paths, ????????save_dir?/?f"val_batch{batch_i}_pred.jpg", ????????names, ????)??#?pred callbacks.run("on_val_batch_end")
3.7 計(jì)算指標(biāo)
指標(biāo)名字在代碼中體現(xiàn)
#Computemetrics stats=[flow.cat(x,0).cpu().numpy()forxinzip(*stats)]#tonumpy iflen(stats)andstats[0].any(): tp,fp,p,r,f1,ap,ap_class=ap_per_class(*stats,plot=plots,save_dir=save_dir,names=names) ap50,ap=ap[:,0],ap.mean(1)#AP@0.5,AP@0.5:0.95 mp,mr,map50,map=p.mean(),r.mean(),ap50.mean(),ap.mean() nt=np.bincount(stats[3].astype(int),minlength=nc)#numberoftargetsperclass
3.8 打印日志
#Printresultsperclass if(verboseor(nc50?and?not?training))?and?nc?>1andlen(stats): fori,cinenumerate(ap_class): LOGGER.info(pf%(names[c],seen,nt[c],p[i],r[i],ap50[i],ap[i])) #Printspeeds t=tuple(x/seen*1e3forxindt)#speedsperimage ifnottraining: shape=(batch_size,3,imgsz,imgsz) LOGGER.info(f"Speed:%.1fmspre-process,%.1fmsinference,%.1fmsNMSperimageatshape{shape}"%t)
3.9 保存驗(yàn)證結(jié)果
#Plots ifplots: confusion_matrix.plot(save_dir=save_dir,names=list(names.values())) callbacks.run("on_val_end") #SaveJSON ifsave_jsonandlen(jdict): w=Path(weights[0]ifisinstance(weights,list)elseweights).stemifweightsisnotNoneelse""#weights anno_json=str(Path(data.get("path","../coco"))/"annotations/instances_val2017.json")#annotationsjson pred_json=str(save_dir/f"{w}_predictions.json")#predictionsjson LOGGER.info(f" EvaluatingpycocotoolsmAP...saving{pred_json}...") withopen(pred_json,"w")asf: json.dump(jdict,f) #try-catch,會(huì)有哪些error """ pycocotools介紹: https://github.com/cocodataset/cocoapi/blob/master/PythonAPI/pycocoEvalDemo.ipynb 嘗試: 使用pycocotools工具計(jì)算loss COCOAPI-http://cocodataset.org/ 失敗error: 直接打印拋出的異常 1.可能沒有安裝pycocotools,但是網(wǎng)絡(luò)有問題,無法實(shí)現(xiàn)自動(dòng)下載。 2.pycocotools包版本有問題 """ try:#https://github.com/cocodataset/cocoapi/blob/master/PythonAPI/pycocoEvalDemo.ipynb check_requirements(["pycocotools"]) frompycocotools.cocoimportCOCO frompycocotools.cocoevalimportCOCOeval anno=COCO(anno_json)#initannotationsapi pred=anno.loadRes(pred_json)#initpredictionsapi eval=COCOeval(anno,pred,"bbox") ifis_coco: eval.params.imgIds=[int(Path(x).stem)forxindataloader.dataset.im_files]#imageIDstoevaluate eval.evaluate() eval.accumulate() eval.summarize() map,map50=eval.stats[:2]#updateresults(mAP@0.5:0.95,mAP@0.5) exceptExceptionase: LOGGER.info(f"pycocotoolsunabletorun:{e}")
3.10 返回結(jié)果
#Returnresults model.float()#fortraining ifnottraining: s=f" {len(list(save_dir.glob('labels/*.txt')))}labelssavedto{save_dir/'labels'}"ifsave_txtelse"" LOGGER.info(f"Resultssavedto{colorstr('bold',save_dir)}{s}") maps=np.zeros(nc)+map fori,cinenumerate(ap_class): maps[c]=ap[i] return(mp,mr,map50,map,*(loss.cpu()/len(dataloader)).tolist()),maps,t
審核編輯:劉清
-
NMS
+關(guān)注
關(guān)注
0文章
9瀏覽量
6054 -
python
+關(guān)注
關(guān)注
56文章
4807瀏覽量
84955 -
JSON
+關(guān)注
關(guān)注
0文章
119瀏覽量
6996 -
解釋器
+關(guān)注
關(guān)注
0文章
103瀏覽量
6551
原文標(biāo)題:《YOLOv5全面解析教程》?十六,val.py 源碼解讀
文章出處:【微信號(hào):GiantPandaCV,微信公眾號(hào):GiantPandaCV】歡迎添加關(guān)注!文章轉(zhuǎn)載請(qǐng)注明出處。
發(fā)布評(píng)論請(qǐng)先 登錄
相關(guān)推薦
Faster Transformer v2.1版本源碼解讀
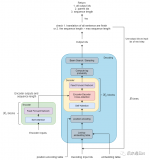
OneFlow Softmax算子源碼解讀之BlockSoftmax
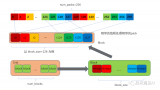
【HarmonyOS IPC 試用連載 】鴻蒙源碼編譯報(bào)錯(cuò) 無法找到build.py的錯(cuò)誤
【HarmonyOS】鴻蒙源碼編譯報(bào)錯(cuò) 無法找到build.py的錯(cuò)誤
AP側(cè)中網(wǎng)相關(guān)的PLMN業(yè)務(wù)源碼流程解讀
51單片機(jī)拼音中文輸入法c程序源代碼
海爾29F3A-PY、29F9B-PY(100赫茲)彩電電路
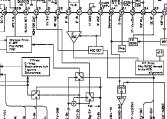
51單片機(jī)的中文拼音輸入法-c語言源程序
基于MCU的U盤源碼實(shí)現(xiàn)flash的windos文件系統(tǒng)
基于EAIDK的人臉?biāo)惴☉?yīng)用-源碼解讀(2)
openharmony源碼解讀
Auto-py-to-exe:將Python腳本轉(zhuǎn)為Exe的流程

評(píng)論