什么是pass?
Pass是TVM中基于relay IR進行的優化,目的是去除冗余算子,進行硬件友好的算子轉換,最終能夠提高硬件運行效率。由tensorflow等深度學習框架生成的圖機構中,含有很多可以優化的算子,比如expand_dim,len等,其實在編譯階段完全可以優化掉,從而能夠減少硬件的計算,以及避免出現硬件不支持的算子。
TVM中在include/tvm/ir/transform.h中對pass進行了抽象,主要包括PassContext,PassInfo,Pass,以及Sequential。其中PassContext包含了pass執行依賴的一些參數,比如優化level,analysis report等。PassInfo是一個用于記錄pass信息的類,包括pass的opt-level,名稱等。和PassContext的區別是PassContext是pass執行所需要獲取的條件。Pass就是執行pass的主體,主要就是pass的函數。比如RemoveUnusedFunctions就是執行pass的一個主體函數,目的就是去除冗余算子。Sequential是一個container,裝載所有pass。
一些pass
01. RemoveUnusedFunctions
位于src/relay/backend/vm/removed_unused_funcs.cc中,顧名思義就是去除relay IR中的冗余函數。通過從main函數開始遍歷,如果一個函數體沒有引用其它函數,而同時又沒有被其它函數調用,即從relay圖上看是一個孤立算子,那么就從IRModule中刪除。
void VisitExpr_(const FunctionNode* func_node) final { auto func = GetRef(func_node); if (visiting_.find(func) == visiting_.end()) { visiting_.insert(func); for (auto param : func_node->params) { ExprVisitor::VisitExpr(param); } ExprVisitor::VisitExpr(func_node-> body); } }
02. ToBasicBlockNormalForm
函數在文件src/relay/trnaforms/to_basic_block_normal_from.cc中。通過遍歷IRModule中的每個function,將每個function轉換為基本塊形式。轉換函數是ToBasicBlockNormalFormAux。這個函數包括兩個步驟:一是找到基本塊(basic block)的邊界,TVM中對邊界進行了一步抽象,判斷每個expr是否屬于同一個scope,如果scope相同那么就可以將這些表達式放在一個基本塊中;第二步根據每個表達式所屬的scope將表達式歸屬到一個基本塊中。
Expr ToBasicBlockNormalFormAux(const Expr& e) { // calculate all the dependency between nodes. support::Arena arena; DependencyGraph dg = DependencyGraph::Create(&arena, e); /* The scope of the whole expr is global. * The scope of any subexpr, is the lowest common ancestor of all incoming edge. * We also record the set of expressions whose scope is lifted. */ std::pair scopes = CalcScope(dg); return Fill::ToBasicBlockNormalForm(e, dg, &scopes.first, &scopes.second); }
DependencyGraph是一個表達式相互依賴的圖結構,通過遍歷圖中每個節點,找到每個節點的scope。CalcScope在文件src/relay/transforms/to_a_normal_from.cc中。這個函數中重點關注以下代碼:
… s = LCA(s, expr_scope.at(iit->value)); … if (n->new_scope) { auto child_scope = std::make_shared(s); expr_scope.insert({n, child_scope}); } else { expr_scope.insert({n, s}); }
LCA是獲得當前節點的父節點的scope的LCA(least common ancestor),然后將這個scope作為這個節點的scope。了解基本塊原理的都知道,尋找基本塊首先要找到首指令的位置,然后一個首指令到下一個首指令之間的指令就屬于一個基本塊。而首指令就是那些具有條件和無條件跳轉的指令。在TVM中通過new_scope來標記這些節點,比如Ifnode,FunctionNode,LetNode在建立dependency圖的時候,這些節點就被標記為new_scope。這樣就建立了dependency節點到scope節點的對應map。同時scope節點也被建立起樹結構。
接下來就是建立Fill類,這個類中包含了dependency圖以及scope的信息,通過其函數ToBasicBlockNormalForm實現基本塊轉換。它的基本邏輯通過VisitExpr函數遍歷dependency節點,將具有相同scope的節點壓入到同一個let_list中。Let_list文檔中是這樣解釋的:
/*! * \file let_list.h * \brief LetList record let binding and insert let expression implicitly. * using it, one can treat AST as value instead of expression, * and pass them around freely without fear of AST explosion (or effect duplication). * for example, if one write 'b = a + a; c = b + b; d = c + c', the AST will contain 8 'a'. * if one instead write 'b = ll.Push(a + a); c = ll.Push(b + b); d = ll.Get(c + c);', * the AST will contain 2 'a', as b and c are now variables.
Let_list使得抽象語法樹簡潔化,不會因為變量的復制導致樹的爆炸。具有相同的scope的expr被約束到相同的let_list中,用一個var來表達,這樣就將表達式轉化為var的形式。一個var也就對應了一個基本塊。
03. Legalize
Legalize是實現等價函數的轉換。主要代碼在src/relay/transforms/legalize.cc中。主函數是:
Expr Legalize(const Expr& expr, const std::string& legalize_map_attr_name) { auto rewriter = Legalizer(legalize_map_attr_name); return PostOrderRewrite(expr, &rewriter); }
在legalize.cc文件中定義了一個繼承了ExprRewriter的類,在這個類中實現了對function的替換。我們追蹤一下調用的過程。PostOrderRewrite在文件src/relay/ir/expr_functor.cc中。首先建立一個PostOrderRewriter類,然后訪問每個節點。在訪問節點過程中調用了ExpandDataFlow函數,看一下這個函數的描述:
* * ExpandDataflow manually manages a stack and performs DFS to determine the processing * order of nodes in an input graph. * * If it finds a dataflow node (Call, Tuple, TupleGetItem), it checks if the arguments to that node * need to be processed via fcheck_visited. If so, the function pushes those arguments to the stack * and continues iteratively to process the top of the stack. When it finds a node that doesn't * match the dataflow types, or a node who's inputs have all been processed, it visits the current * leaf via fvisit_leaf. * * This function should be used internally to other classes to implement mixed-mode traversals. The * expectation is that fvisit_leaf will perform recursive analysis within mixed-mode traversal if it * hits a non-dataflow node. * * fcheck_visited and fvisit_leaf are templated to encourage compiler inlining. */
主要目的是有區別的去處理graph中的節點,如果fcheck_visited已經確定該節點處理過或者不需要處理,就跳過,通過fvisit_leaf繼續訪問下一個節點。而在VisitLeaf函數中就調用了legalizer類中的rewrite_函數實現了legalize功能。在Rewrite_中,通過映射表legalize_map_attr_name實現函數的等價轉換。
04. SimplifyInference
實現對batch normalization, layer normalization, instance normalization, group normalization, L2 normalization算子的分解,這樣做的目的是可以在之后的優化中,將這些算子融合到其它算子上,減少計算量。代碼在src/relay/transforms/simplify_inference.cc中。文件中定義了一個InferenceSimplifier類來處理這個問題。看一下這幾個normalization的公式:
1 BN:
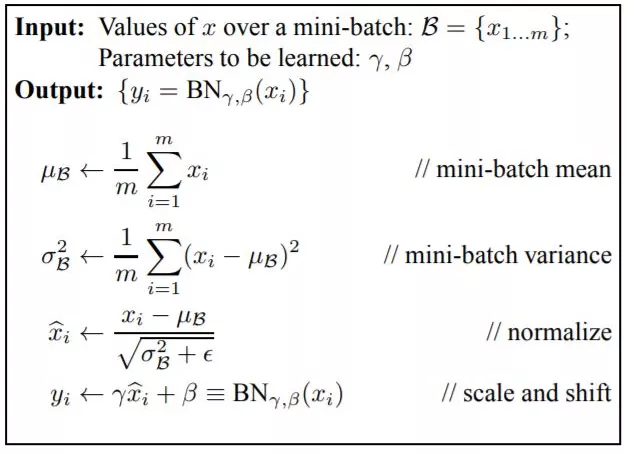
2 LN:獲得均值和方差是基于同一層不同神經元的數據。歸一化公式相同。
3 GN: 將每個輸入樣本沿著通道進行分組,在每個組內進行歸一化。
4 IN:對每個通道的數據進行歸一化。
來看一下bacth normalization的處理代碼:
Expr BatchNormToInferUnpack(const Attrs attrs, Expr data, Expr gamma, Expr beta, Expr moving_mean, Expr moving_var, Type tdata) { auto ttype = tdata.as(); CHECK(ttype); const auto param = attrs.as< BatchNormAttrs>(); Expr epsilon = MakeConstantScalar(ttype->dtype, static_cast(param->epsilon)); Expr var_add_eps = Add(moving_var, epsilon); Expr sqrt_var = Sqrt(var_add_eps); Expr scale = Divide(MakeConstantScalar(ttype->dtype, 1.0f), sqrt_var); if (param->scale) { scale = Multiply(scale, gamma); } Expr neg_mean = Negative(moving_mean); Expr shift = Multiply(neg_mean, scale); if (param->center) { shift = Add(shift, beta); } auto ndim = ttype->shape.size(); int axis = (param->axis < 0) ? param->axis + ndim : param->axis; scale = ExpandBiasToMatchAxis(scale, ndim, {axis}); shift = ExpandBiasToMatchAxis(shift, ndim, {axis}); Expr out = Multiply(data, scale); out = Add(out, shift); return out; }
可以看到就是將batch norm算子分解成最基本的加減乘除算子。
05. EliminateCommonSubexpr
顧名思義,這個pass的目的是消除公共子表達式。公共子表達式類似這種:
a=b+c
d=b+c
兩個表達式具有相同的op,同時又有相同的args,而且args的順序也一樣。那么就可以用一個表達式替換。
這個pass的實現在文件src/relay/transforms/eliminate_common_subexpr.cc中。TVM定義了類CommonSubexprEliminator來處理。重載函數Rewrite_實現了對expr的遍歷和重寫操作。
Expr Rewrite_(const CallNode* call, const Expr& post) final { … if (new_call->args.size() == 0 || op == nullptr || op_stateful.get(GetRef< Op>(op), false)) { return new_expr; } if (fskip_ != nullptr && fskip_(new_expr)) { return new_expr; } auto it = expr_map_.find(new_call->op); if (it != expr_map_.end()) { for (const Expr& candidate_expr : it->second) { if (const CallNode* candidate = candidate_expr.as< CallNode>()) { bool is_equivalent = true; if (!attrs_equal(new_call->attrs, candidate->attrs)) { continue; } for (size_t i = 0; i < new_call->args.size(); i++) { if (!new_call->args[i].same_as(candidate->args[i]) && !IsEqualScalar(new_call->args[i], candidate->args[i])) { is_equivalent = false; break; } } if (!is_equivalent) continue; return GetRef(candidate); } } } expr_map_[new_call->op].push_back(new_expr); return new_expr; }
使用一個expr_map_映射記錄已經遍歷過的具有相同op的expr,之后每次遇到相同的op都會對已經記錄的expr進行匹配,匹配包括attrs以及args,如果二者都一樣的話,證明就是公共子表達式。
沒有看過的pass
以上是實現相對簡單的pass,TVM中還實現了其它很多pass,就沒有一一去讀代碼了。以后看需要再去讀吧。現在做一些羅列:
1 SimplifyExpr
簡化一些表達式,具體如何進行簡化需要讀代碼了。
2 CombineParallelConv2D
合并多分支并行的conv2d運算,理解是對多個batch的conv2d進行合并。
3 CombineParalleleDense
將多個batch的dense操作合并為一個batch_matmul操作。
4 CombineParallelBatchMatmul
對多個并行的batch_mamul再進行合并。
這幾個combine操作可能是針對GPU器件的一個多數據并行性的優化。
5 FoldConstant
典型的一個常量合并優化。
6 FoldScaleAxis
包含了ForwardFoldScaleAxis和backwardFoldScaleAxis,主要是將scale參數合并到conv/dense操作的權重參數中。
7 CanonicalizeCast
官方解釋是: Canonicalize cast expressions to make operator fusion more efficient。理解是對一些cast操作規范化,就是讓復雜的cast操作可以更簡潔。
8 CanonicalizeOps
規范化一些算子,比如bias_add能夠被表示為expand_dims和broadcast_add操作。
審核編輯 黃昊宇
…
-
優化
+關注
關注
0文章
220瀏覽量
23945 -
TVM
+關注
關注
0文章
19瀏覽量
3683
發布評論請先 登錄
相關推薦
TVM主要的編譯過程解析
TVM整體結構,TVM代碼的基本構成
將TVM用于移動端常見的ARM GPU,提高移動設備對深度學習的支持能力
什么是波場虛擬機TVM
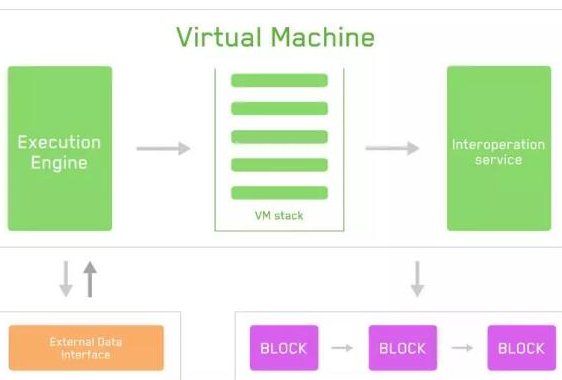
Linux的基礎學習筆記資料總結
TVM的編譯流程是什么
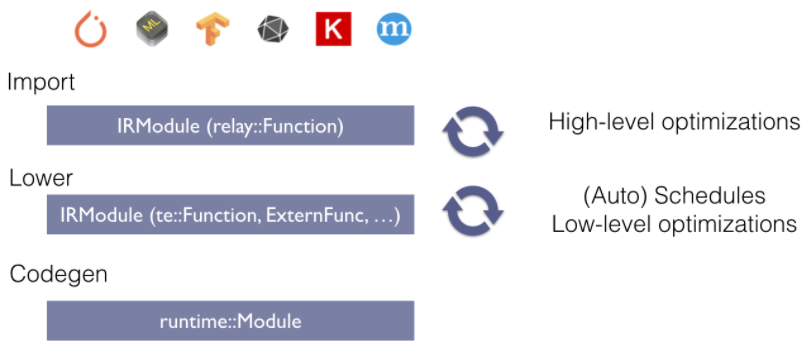
TVM學習(三)編譯流程
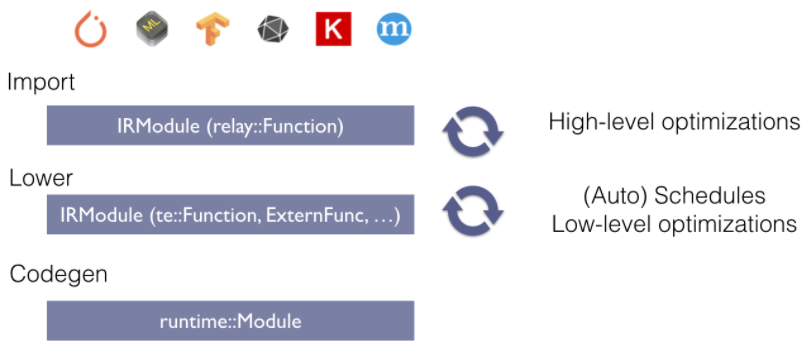
TVM學習(二):算符融合
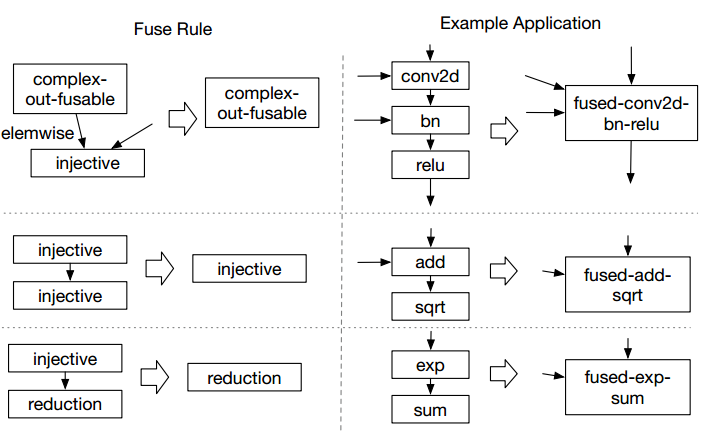
使用TVM在android中進行Mobilenet SSD部署
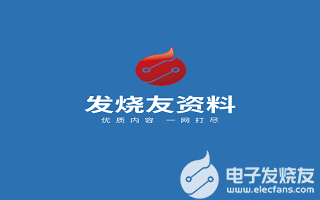
評論