神經(jīng)架構(gòu)學習 (Neural Structured Learning,NSL) 是 TensorFlow 中的一個框架,可以利用結(jié)構(gòu)化信號來訓練神經(jīng)網(wǎng)絡。這種框架接受 (i) 顯式計算圖或 (ii) 隱式計算圖,處理結(jié)構(gòu)化輸入,并在模型訓練過程中動態(tài)生成鄰接點(Neighbors)。顯式計算圖的 NSL 通常用于基于神經(jīng)網(wǎng)絡的圖學習(Neural Graph Learning),而隱式計算圖的 NSL 通常用于 對抗學習。這兩種技術(shù)均以 NSL 框架中的正則化形式實現(xiàn)。所以,它們只對訓練工作流有影響,而工作流的模型保持不變。我們將在本文中探討如何在 TFX 中使用 NSL 框架實現(xiàn)計算圖正則化(Graph Regularization )。
神經(jīng)架構(gòu)學習
https://tensorflow.google.cn/neural_structured_learning
使用 NSL 構(gòu)建計算圖正則化模型的高級工作流包含以下步驟:
如果沒有可用的計算圖,則需要先構(gòu)建一個計算圖。
使用計算圖和輸入樣本特征擴充訓練數(shù)據(jù)。
使用擴充的訓練數(shù)據(jù)對給定模型進行計算圖正則化。
這些步驟不會立即映射到現(xiàn)有的 TFX (TensorFlow Extended) 流水線組件上。但是,TFX 支持自定義組件,允許用戶在其 TFX 流水線中實現(xiàn)自定義處理。如需了解 TFX 中的自定義組件,請參閱這篇文章。
TFX
https://tensorflow.google.cn/tfx/guide#tfx_standard_components
自定義組件
https://tensorflow.google.cn/tfx/guide/understanding_custom_components
為了在 TFX 中創(chuàng)建一個包含上述步驟的計算圖正則化模型,我們將利用擴展自定義 TFX 組件。
為展示如何使用 NSL,我們構(gòu)建了一個示例 TFX 流水線,對 IMDB 數(shù)據(jù)集進行情感分類。我們提供了一個基于 Colab 的教程,演示了如何使用 NSL 與原生 TensorFlow 來完成這項任務,我們以此作為示例 TFX 流水線的基礎(chǔ)。
IMDB 數(shù)據(jù)集
https://tensorflow.google.cn/datasets/catalog/imdb_reviews
Colab 教程
https://tensorflow.google.cn/neural_structured_learning/tutorials/graph_keras_lstm_imdb
自定義 TFX 組件的計算圖正則化
為了在 TFX 中構(gòu)建一個計算圖正則化的 NSL 模型來完成這項任務,我們將使用自定義 Python 函數(shù)方法自定義三個組件。以下是使用這些自定義組件實現(xiàn)我們示例的 TFX 流水線示意圖。為了簡潔起見,我們省略了通常在 Trainer 之后的組件,例如 Evaluator、 Pusher 等。
自定義 Python 函數(shù)
https://tensorflow.google.cn/tfx/guide/custom_function_component
圖 1:TFX 流水線示例:使用計算圖正則化進行文本分類
在此圖中,僅有自定義組件(粉色)與計算圖正則化的 Trainer組件具備 NSL 相關(guān)邏輯。值得注意的是,此處展示的自定義組件僅作例證,還可以通過其他方式構(gòu)建類似功能的流水線。接下來,我們進一步詳細描述各個自定義組件,并展示相應的代碼段。
IdentifyExamples
此自定義組件為每個訓練樣本分配一個唯一的 ID,將每個訓練樣本與其在計算圖中相應的鄰接點關(guān)聯(lián)起來。
@component def IdentifyExamples( orig_examples: InputArtifact[Examples], identified_examples: OutputArtifact[Examples], id_feature_name: Parameter[str], component_name: Parameter[str] ) -> None: # Compute the input and output URIs. ... # For each input split, update the TF.Examples to include a unique ID. with beam.Pipeline() as pipeline: (pipeline | 'ReadExamples' >> beam.io.ReadFromTFRecord( os.path.join(input_dir, '*'), coder=beam.coders.coders.ProtoCoder(tf.train.Example)) | 'AddUniqueId' >> beam.Map(make_example_with_unique_id, id_feature_name) | 'WriteIdentifiedExamples' >> beam.io.WriteToTFRecord( file_path_prefix=os.path.join(output_dir, 'data_tfrecord'), coder=beam.coders.coders.ProtoCoder(tf.train.Example), file_name_suffix='.gz')) identified_examples.split_names = orig_examples.split_names return
make_example_with_unique_id() 函數(shù)可以更新給定樣本,將包含唯一 ID 的額外特征包括在內(nèi)。
SynthesizeGraph
如上所述,在 IMDB 數(shù)據(jù)集中,沒有提供顯式計算圖作為輸入。因此,在演示計算圖正則化之前,我們將構(gòu)建一個計算圖。在此示例中,我們使用一個預訓練的文本嵌入向量模型將電影評論中的原始文本轉(zhuǎn)換為嵌入向量,然后通過生成的嵌入向量構(gòu)建計算圖。
SynthesizeGraph自定義組件負責處理計算圖構(gòu)建,請注意,它定義了一個新的Artifact,名為 SynthesizedGraph,作為此自定義組件的輸出。
"""Custom Artifact type""" class SynthesizedGraph(tfx.types.artifact.Artifact): """Output artifact of the SynthesizeGraph component""" TYPE_NAME = 'SynthesizedGraphPath' PROPERTIES = { 'span': standard_artifacts.SPAN_PROPERTY, 'split_names': standard_artifacts.SPLIT_NAMES_PROPERTY, } @component def SynthesizeGraph( identified_examples: InputArtifact[Examples], synthesized_graph: OutputArtifact[SynthesizedGraph], similarity_threshold: Parameter[float], component_name: Parameter[str] ) -> None: # Compute the input and output URIs ... # We build a graph only based on the 'train' split which includes both # labeled and unlabeled examples. create_embeddings(train_input_examples_uri, output_graph_uri) build_graph(output_graph_uri, similarity_threshold) synthesized_graph.split_names = artifact_utils.encode_split_names( splits=['train']) return
create_embeddings() 函數(shù)通過 TensorFlow Hub 上的一些預訓練模型將電影評論中的文本轉(zhuǎn)換為相應的嵌入向量。build_graph() 函數(shù)調(diào)用 NSL 中的 build_graph()API。
TensorFlow Hub
https://tensorflow.google.cn/hub
build_graph()
https://tensorflow.google.cn/neural_structured_learning/api_docs/python/nsl/tools/build_graph
GraphAugmentation
此自定義組件的目的在于將樣本特征(電影評論中的文本)與通過嵌入向量構(gòu)建的計算圖結(jié)合起來,生成一個擴充的訓練數(shù)據(jù)集。由此得出的訓練樣本也將包括其相應鄰接點的特征。
@component def GraphAugmentation( identified_examples: InputArtifact[Examples], synthesized_graph: InputArtifact[SynthesizedGraph], augmented_examples: OutputArtifact[Examples], num_neighbors: Parameter[int], component_name: Parameter[str] ) -> None: # Compute the input and output URIs ... # Separate out the labeled and unlabeled examples from the 'train' split. train_path, unsup_path = split_train_and_unsup(train_input_uri) # Augment training data with neighbor features. nsl.tools.pack_nbrs( train_path, unsup_path, graph_path, output_path, add_undirected_edges=True, max_nbrs=num_neighbors ) # Copy the 'test' examples from input to output without modification. ... augmented_examples.split_names = identified_examples.split_names return
split_train_and_unsup() 函數(shù)將輸入樣本拆分成帶標簽和無標簽的樣本,pack_nbrs() NSL API 創(chuàng)建擴充的訓練數(shù)據(jù)集。
pack_nbrs()
https://tensorflow.google.cn/neural_structured_learning/api_docs/python/nsl/tools/pack_nbrs
計算圖正則化的 Trainer
我們目前所有的自定義組件都已實現(xiàn),TFX 流水線的Trainer 組件中增加了其他 NSL 相關(guān)的內(nèi)容。下方展示了一個計算圖正則化 Trainer 組件的簡化視圖。
... estimator = tf.estimator.Estimator( model_fn=feed_forward_model_fn, config=run_config, params=HPARAMS) # Create a graph regularization config. graph_reg_config = nsl.configs.make_graph_reg_config( max_neighbors=HPARAMS.num_neighbors, multiplier=HPARAMS.graph_regularization_multiplier, distance_type=HPARAMS.distance_type, sum_over_axis=-1) # Invoke the Graph Regularization Estimator wrapper to incorporate # graph-based regularization for training. graph_nsl_estimator = nsl.estimator.add_graph_regularization( estimator, embedding_fn, optimizer_fn=optimizer_fn, graph_reg_config=graph_reg_config) ...
如您所見,創(chuàng)建了基礎(chǔ)模型后(本例中指一個前饋神經(jīng)網(wǎng)絡),就可以通過調(diào)用 NSL 封裝容器 API 將其直接轉(zhuǎn)換為計算圖正則化模型。
一切就這么簡單。現(xiàn)在,我們已補充完在 TFX 中構(gòu)建計算圖正則化 NSL 模型所需的步驟。此處提供了一個基于 Colab 的教程,在 TFX 中端到端地演示這個示例。不妨嘗試一下,并可根據(jù)您的需要進行自定義。
此處
https://tensorflow.google.cn/tfx/tutorials/tfx/neural_structured_learning
對抗學習
如前文簡介中所述,神經(jīng)架構(gòu)學習的另一個方面是對抗學習,即不使用計算圖中的顯式鄰接點來進行正則化,而是動態(tài)、對抗性地創(chuàng)建隱式鄰接點來迷惑模型。
因此,使用對抗樣本進行正則化是提高模型魯棒性的有效方式。使用神經(jīng)架構(gòu)學習的對抗學習可以輕松集成到 TFX 流水線中。無需任何自定義組件,只需要更新 Trainer 組件即可在神經(jīng)架構(gòu)學習中調(diào)用對抗正則化封裝容器 API。
總結(jié)
我們演示了如何利用自定義組件在 TFX 中使用神經(jīng)架構(gòu)學習構(gòu)建計算圖正則化模型。當然,也可以用其他方式構(gòu)建計算圖,或者按照另外的方式來構(gòu)建整體流水線。
我們希望這個示例能夠為您構(gòu)建自己的神經(jīng)架構(gòu)學習工作流提供幫助。
相關(guān)鏈接
有關(guān)神經(jīng)架構(gòu)學習的更多信息,請查閱以下資源:
TFX 中的 NSL Colab 教程
https://tensorflow.google.cn/tfx/tutorials/tfx/neural_structured_learning
NSL 網(wǎng)站
https://tensorflow.google.cn/neural_structured_learning
NSL GitHub
https://github.com/tensorflow/neural-structured-learning
更多 NSL 教程與視頻
https://github.com/tensorflow/neural-structured-learning#videos-and-colab-tutorials
致謝:
我們特此鳴謝 Google 神經(jīng)架構(gòu)學習團隊、TFX 團隊以及 Aurélien Geron 的支持與貢獻。
責任編輯:xj
原文標題:TensorFlow Extended + 神經(jīng)架構(gòu)學習:構(gòu)建計算圖正則化模型
文章出處:【微信公眾號:TensorFlow】歡迎添加關(guān)注!文章轉(zhuǎn)載請注明出處。
-
神經(jīng)網(wǎng)絡
+關(guān)注
關(guān)注
42文章
4778瀏覽量
101014 -
計算圖
+關(guān)注
關(guān)注
0文章
9瀏覽量
6934 -
正則化
+關(guān)注
關(guān)注
0文章
17瀏覽量
8144 -
tensorflow
+關(guān)注
關(guān)注
13文章
329瀏覽量
60583
原文標題:TensorFlow Extended + 神經(jīng)架構(gòu)學習:構(gòu)建計算圖正則化模型
文章出處:【微信號:tensorflowers,微信公眾號:Tensorflowers】歡迎添加關(guān)注!文章轉(zhuǎn)載請注明出處。
發(fā)布評論請先 登錄
相關(guān)推薦
如何在Windows中使用MTP協(xié)議
如何在智能手機系統(tǒng)中使用bq27505
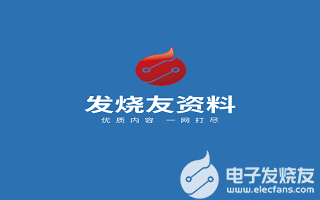
如何在MSP430?MCU中使用智能模擬組合
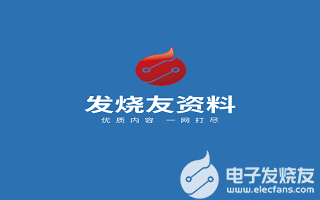
如何在反向降壓-升壓拓撲中使用TPS6290x
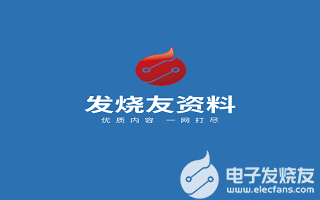
如何在汽車CAN應用中使用負邊緣觸發(fā)觸發(fā)器節(jié)省電力
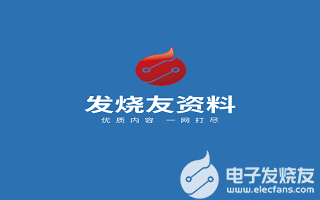
如何在新興的低軌衛(wèi)星應用中使用數(shù)字隔離器隔離信號
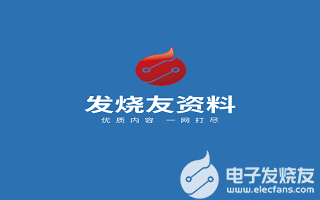
深度學習模型中的過擬合與正則化
如何在IDF框架中使用自定義的靜態(tài)庫和動態(tài)庫?
請問cmakelists中的變量如何在程序中使用?
鴻蒙Ability Kit(程序框架服務)【應用啟動框架AppStartup】
工業(yè)計算機是什么?如何在不同行業(yè)中使用?
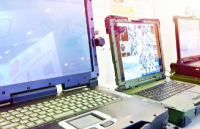
評論