作者:Lianne & Justin? ?
在擬合機器學習或統(tǒng)計模型之前,我們通常需要清洗數(shù)據(jù)。用雜亂數(shù)據(jù)訓練出的模型無法輸出有意義的結(jié)果。
數(shù)據(jù)清洗:從記錄集、表或數(shù)據(jù)庫中檢測和修正(或刪除)受損或不準確記錄的過程。它識別出數(shù)據(jù)中不完善、不準確或不相關(guān)的部分,并替換、修改或刪除這些臟亂的數(shù)據(jù)。
「數(shù)據(jù)清洗」光定義就這么長,執(zhí)行過程肯定既枯燥又耗時。 ? ? ? ? 為了將數(shù)據(jù)清洗簡單化,本文介紹了一種新型完備分步指南,支持在 Python 中執(zhí)行數(shù)據(jù)清洗流程。讀者可以學習找出并清洗以下數(shù)據(jù)的方法:
缺失數(shù)據(jù);
不規(guī)則數(shù)據(jù)(異常值);
不必要數(shù)據(jù):重復數(shù)據(jù)(repetitive data)、復制數(shù)據(jù)(duplicate data)等;
不一致數(shù)據(jù):大寫、地址等;
該指南使用的數(shù)據(jù)集是 Kaggle 競賽 Sberbank 俄羅斯房地產(chǎn)價值預測競賽數(shù)據(jù)(該項目的目標是預測俄羅斯的房價波動)。本文并未使用全部數(shù)據(jù),僅選取了其中的一部分樣本。 ? ? ? ? 在進入數(shù)據(jù)清洗流程之前,我們先來看一下數(shù)據(jù)概況。
# import packages import pandas as pd import numpy as np import seaborn as sns import matplotlib.pyplot as plt import matplotlib.mlab as mlab import matplotlib plt.style.use('ggplot') from matplotlib.pyplot import figure %matplotlib inline matplotlib.rcParams['figure.figsize'] = (12,8) pd.options.mode.chained_assignment = None # read the data df = pd.read_csv('sberbank.csv') # shape and data types of the data print(df.shape) print(df.dtypes) # select numeric columns df_numeric = df.select_dtypes(include=[np.number]) numeric_cols = df_numeric.columns.values print(numeric_cols) # select non numeric columns df_non_numeric = df.select_dtypes(exclude=[np.number]) non_numeric_cols = df_non_numeric.columns.values print(non_numeric_cols)
cols = df.columns[:30] # first 30 columns colours = ['#000099', '#ffff00'] # specify the colours - yellow is missing. blue is not missing. sns.heatmap(df[cols].isnull(), cmap=sns.color_palette(colours))下表展示了前 30 個特征的缺失數(shù)據(jù)模式。橫軸表示特征名,縱軸表示觀察值/行數(shù),黃色表示缺失數(shù)據(jù),藍色表示非缺失數(shù)據(jù)。 ? 例如,下圖中特征 life_sq 在多個行中存在缺失值。而特征 floor 只在第 7000 行左右出現(xiàn)零星缺失值。 ?
# if it's a larger dataset and the visualization takes too long can do this. # % of missing. for col in df.columns: pct_missing = np.mean(df[col].isnull()) print('{} - {}%'.format(col, round(pct_missing*100)))? 得到如下列表,該表展示了每個特征的缺失值百分比。 ? 具體而言,我們可以從下表中看到特征 life_sq 有 21% 的缺失數(shù)據(jù),而特征 floor 僅有 1% 的缺失數(shù)據(jù)。該列表有效地總結(jié)了每個特征的缺失數(shù)據(jù)百分比情況,是對熱圖可視化的補充。 ?
# first create missing indicator for features with missing data for col in df.columns: missing = df[col].isnull() num_missing = np.sum(missing) if num_missing > 0: print('created missing indicator for: {}'.format(col)) df['{}_ismissing'.format(col)] = missing # then based on the indicator, plot the histogram of missing values ismissing_cols = [col for col in df.columns if 'ismissing' in col] df['num_missing'] = df[ismissing_cols].sum(axis=1) df['num_missing'].value_counts().reset_index().sort_values(by='index').plot.bar(x='index', y='num_missing')? 直方圖可以幫助在 30,471 個觀察值中識別缺失值狀況。 ? 例如,從下圖中可以看到,超過 6000 個觀察值不存在缺失值,接近 4000 個觀察值具備一個缺失值。 ?
# drop rows with a lot of missing values. ind_missing = df[df['num_missing'] > 35].index df_less_missing_rows = df.drop(ind_missing, axis=0)? 解決方案 2:丟棄特征 ? 與解決方案 1 類似,我們只在確定某個特征無法提供有用信息時才丟棄它。 ? 例如,從缺失數(shù)據(jù)百分比列表中,我們可以看到 hospital_beds_raion 具備較高的缺失值百分比——47%,因此我們丟棄這一整個特征。 ?
# hospital_beds_raion has a lot of missing. # If we want to drop. cols_to_drop = ['hospital_beds_raion'] df_less_hos_beds_raion = df.drop(cols_to_drop, axis=1)? 解決方案 3:填充缺失數(shù)據(jù) ? 當特征是數(shù)值變量時,執(zhí)行缺失數(shù)據(jù)填充。對同一特征的其他非缺失數(shù)據(jù)取平均值或中位數(shù),用這個值來替換缺失值。 ? 當特征是分類變量時,用眾數(shù)(最頻值)來填充缺失值。 ? 以特征 life_sq 為例,我們可以用特征中位數(shù)來替換缺失值。 ?
# replace missing values with the median. med = df['life_sq'].median() print(med) df['life_sq'] = df['life_sq'].fillna(med)? 此外,我們還可以對所有數(shù)值特征一次性應用同樣的填充策略。 ?
# impute the missing values and create the missing value indicator variables for each numeric column. df_numeric = df.select_dtypes(include=[np.number]) numeric_cols = df_numeric.columns.values for col in numeric_cols: missing = df[col].isnull() num_missing = np.sum(missing) if num_missing > 0: # only do the imputation for the columns that have missing values. print('imputing missing values for: {}'.format(col)) df['{}_ismissing'.format(col)] = missing med = df[col].median() df[col] = df[col].fillna(med)?
# impute the missing values and create the missing value indicator variables for each non-numeric column. df_non_numeric = df.select_dtypes(exclude=[np.number]) non_numeric_cols = df_non_numeric.columns.values for col in non_numeric_cols: missing = df[col].isnull() num_missing = np.sum(missing) if num_missing > 0: # only do the imputation for the columns that have missing values. print('imputing missing values for: {}'.format(col)) df['{}_ismissing'.format(col)] = missing top = df[col].describe()['top'] # impute with the most frequent value. df[col] = df[col].fillna(top)
解決方案 4:替換缺失值 ? 對于分類特征,我們可以添加新的帶值類別,如 _MISSING_。對于數(shù)值特征,我們可以用特定值(如-999)來替換缺失值。 ? 這樣,我們就可以保留缺失值,使之提供有價值的信息。??
# categorical df['sub_area'] = df['sub_area'].fillna('_MISSING_') # numeric df['life_sq'] = df['life_sq'].fillna(-999)? 不規(guī)則數(shù)據(jù)(異常值) ? 異常值指與其他觀察值具備顯著差異的數(shù)據(jù),它們可能是真的異常值也可能是錯誤。 ? 如何找出異常值? ? 根據(jù)特征的屬性(數(shù)值或分類),使用不同的方法來研究其分布,進而檢測異常值。 ? 方法 1:直方圖/箱形圖 ? 當特征是數(shù)值變量時,使用直方圖和箱形圖來檢測異常值。 ? 下圖展示了特征 life_sq 的直方圖。 ?
# histogram of life_sq. df['life_sq'].hist(bins=100)? 由于數(shù)據(jù)中可能存在異常值,因此下圖中數(shù)據(jù)高度偏斜。 ?
# box plot. df.boxplot(column=['life_sq'])? 從下圖中我們可以看到,異常值是一個大于 7000 的數(shù)值。 ?
df['life_sq'].describe()?
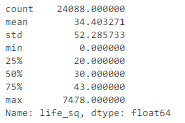
# bar chart?-? distribution of a categorical variable df['ecology'].value_counts().plot.bar()?
num_rows = len(df.index) low_information_cols = [] # for col in df.columns: cnts = df[col].value_counts(dropna=False) top_pct = (cnts/num_rows).iloc[0] if top_pct > 0.95: low_information_cols.append(col) print('{0}: {1:.5f}%'.format(col, top_pct*100)) print(cnts) print()? 我們可以逐一查看這些變量,確認它們是否提供有用信息。(此處不再詳述。) ?
# we know that column 'id' is unique, but what if we drop it? df_dedupped = df.drop('id', axis=1).drop_duplicates() # there were duplicate rows print(df.shape) print(df_dedupped.shape)? 我們發(fā)現(xiàn),有 10 行是完全復制的觀察值。 ?

key = ['timestamp', 'full_sq', 'life_sq', 'floor', 'build_year', 'num_room', 'price_doc'] df.fillna(-999).groupby(key)['id'].count().sort_values(ascending=False).head(20)? 基于這組關(guān)鍵特征,我們找到了 16 條復制數(shù)據(jù)。 ?
# drop duplicates based on an subset of variables. key = ['timestamp', 'full_sq', 'life_sq', 'floor', 'build_year', 'num_room', 'price_doc'] df_dedupped2 = df.drop_duplicates(subset=key) print(df.shape) print(df_dedupped2.shape)? 刪除 16 條復制數(shù)據(jù),得到新數(shù)據(jù)集 df_dedupped2。 ?

df['sub_area'].value_counts(dropna=False)? 它存儲了不同地區(qū)的名稱,看起來非常標準化。 ?
# make everything lower case. df['sub_area_lower'] = df['sub_area'].str.lower() df['sub_area_lower'].value_counts(dropna=False)?
df?
df['timestamp_dt'] = pd.to_datetime(df['timestamp'], format='%Y-%m-%d') df['year'] = df['timestamp_dt'].dt.year df['month'] = df['timestamp_dt'].dt.month df['weekday'] = df['timestamp_dt'].dt.weekday print(df['year'].value_counts(dropna=False)) print() print(df['month'].value_counts(dropna=False))?
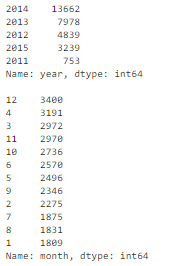
from nltk.metrics import edit_distance df_city_ex = pd.DataFrame(data={'city': ['torontoo', 'toronto', 'tronto', 'vancouver', 'vancover', 'vancouvr', 'montreal', 'calgary']}) df_city_ex['city_distance_toronto'] = df_city_ex['city'].map(lambda x: edit_distance(x, 'toronto')) df_city_ex['city_distance_vancouver'] = df_city_ex['city'].map(lambda x: edit_distance(x, 'vancouver')) df_city_ex?
msk = df_city_ex['city_distance_toronto'] <= 2 df_city_ex.loc[msk, 'city'] = 'toronto' msk = df_city_ex['city_distance_vancouver'] <= 2 df_city_ex.loc[msk, 'city'] = 'vancouver' df_city_ex?
# no address column in the housing dataset. So create one to show the code. df_add_ex = pd.DataFrame(['123 MAIN St Apartment 15', '123 Main Street Apt 12 ', '543 FirSt Av', ' 876 FIRst Ave.'], columns=['address']) df_add_ex? 我們可以看到,地址特征非常混亂。 ?
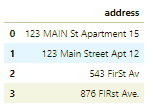
df_add_ex['address_std'] = df_add_ex['address'].str.lower() df_add_ex['address_std'] = df_add_ex['address_std'].str.strip() # remove leading and trailing whitespace. df_add_ex['address_std'] = df_add_ex['address_std'].str.replace('\.', '') # remove period. df_add_ex['address_std'] = df_add_ex['address_std'].str.replace('\bstreet\b', 'st') # replace street with st. df_add_ex['address_std'] = df_add_ex['address_std'].str.replace('\bapartment\b', 'apt') # replace apartment with apt. df_add_ex['address_std'] = df_add_ex['address_std'].str.replace('\bav\b', 'ave') # replace apartment with apt. df_add_ex? 現(xiàn)在看起來好多了: ?
編輯:黃飛
?
評論