作者:劉健健
來自:ChallengeHub
Twitter 的推文有許多特點,首先,與 Facebook 不同的是,推文是基于文本的,可以通過 Twitter 接口注冊下載,便于作為自然語言處理所需的語料庫。其次,Twitter 規定了每一個推文不超過 140 個字,實際推文中的文本長短不一、長度一般較短,有些只有一個句子甚至一個短語,這對其開展情感分類標注帶來許多困難。再者,推文常常是隨性所作,內容中包含情感的元素較多,口語化內容居多,縮寫隨處都在,并且使用了許多網絡用語,情緒符號、新詞和俚語隨處可見。因此,與正式文本非常不同。如果采用那些適合處理正式文本的情感分類方法來對 Twitter 推文進行情感分類,效果將不盡人意。
公眾情感在包括電影評論、消費者信心、政治選舉、股票走勢預測等眾多領域發揮著越來越大的影響力。面向公共媒體內容開展情感分析是分析公眾情感的一項基礎工作。
二、數據基本情況
數據集基于推特用戶發表的推文數據集,并且針對部分字段做出了一定的調整,所有的字段信息請以本練習賽提供的字段信息為準
字段信息內容參考如下:
tweet_id string 推文數據的唯一ID,比如test_0,train_1024
content string 推特內容
label int 推特情感的類別,共13種情感
其中訓練集train.csv包含3w條數據,字段包括tweet_id,content,label;測試集test.csv包含1w條數據,字段包括tweet_id,content。
tweet_id,content,label
tweet_1,Layinnbedwithaheadacheughhhh...waitinonyourcall...,1
tweet_2,Funeralceremony...gloomyfriday...,1
tweet_3,wantstohangoutwithfriendsSOON!,2
tweet_4,"@dannycastilloWewanttotradewithsomeonewhohasHoustontickets,butnoonewill.",3
tweet_5,"Ishouldbesleep,butimnot!thinkingaboutanoldfriendwhoIwant.buthe'smarriednow.damn,&hewantsme2!scandalous!",1
tweet_6,Hmmm.
http://www.djhero.com/isdown,4
tweet_7,@charvirayCharlenemylove.Imissyou,1
tweet_8,cantfallasleep,3
!head/home/mw/input/Twitter4903/train.csv
tweet_id,content,label
tweet_0,@tiffanylueiknowiwaslistenintobadhabitearlierandistartedfreakinathispart=[,0
tweet_1,Layinnbedwithaheadacheughhhh...waitinonyourcall...,1
tweet_2,Funeralceremony...gloomyfriday...,1
tweet_3,wantstohangoutwithfriendsSOON!,2
tweet_4,"@dannycastilloWewanttotradewithsomeonewhohasHoustontickets,butnoonewill.",3
tweet_5,"Ishouldbesleep,butimnot!thinkingaboutanoldfriendwhoIwant.buthe'smarriednow.damn,&hewantsme2!scandalous!",1
tweet_6,Hmmm.http://www.djhero.com/isdown,4
tweet_7,@charvirayCharlenemylove.Imissyou,1
tweet_8,cantfallasleep,3
!head/home/mw/input/Twitter4903/test.csv
tweet_id,content
tweet_0,Re-pinging@ghostridah14:whydidn'tyougotoprom?BCmybfdidn'tlikemyfriends
tweet_1,@kelcouchI'msorryatleastit'sFriday?
tweet_2,Thestormishereandtheelectricityisgone
tweet_3,Sosleepyagainandit'snoteventhatlate.Ifailonceagain.
tweet_4,"WonderingwhyI'mawakeat7am,writinganewsong,plottingmyevilsecretplotsmuahahaha...ohdamnit,notsecretanymore"
tweet_5,IateSomethingIdon'tknowwhatitis...WhydoIkeepTellingthingsaboutfood
tweet_6,sotiredandithinki'mdefinitelygoingtogetanearinfection.goingtobed"early"foronce.
tweet_7,Itissoannoyingwhenshestartstypingonhercomputerinthemiddleofthenight!
tweet_8,Screwyou@davidbrussee!Ionlyhave3weeks...
!head/home/mw/input/Twitter4903/submission.csv
tweet_id,label
tweet_0,0
tweet_1,0
tweet_2,0
tweet_3,0
tweet_4,0
tweet_5,0
tweet_6,0
tweet_7,0
tweet_8,0
三、數據集定義
1.環境準備
環境準備 (建議gpu環境,速度好。pip install paddlepaddle-gpu)
!pipinstallpaddlepaddle
!pipinstall-Upaddlenlp
2.獲取句子最大長度
自定義PaddleNLP dataset的read方法
importpandasaspd
train=pd.read_csv('/home/mw/input/Twitter4903/train.csv')
test=pd.read_csv('/home/mw/input/Twitter4903/test.csv')
sub=pd.read_csv('/home/mw/input/Twitter4903/submission.csv')
print('最大內容長度%d'%(max(train['content'].str.len())))
最大內容長度 166
3.定義數據集
定義讀取函數
defread(pd_data):
forindex,iteminpd_data.iterrows():
yield{'text':item['content'],'label':item['label'],'qid':item['tweet_id'].strip('tweet_')}
分割訓練集、測試機
frompaddle.ioimportDataset,Subset
frompaddlenlp.datasetsimportMapDataset
frompaddlenlp.datasetsimportload_dataset
dataset=load_dataset(read,pd_data=train,lazy=False)
dev_ds=Subset(dataset=dataset,indices=[iforiinrange(len(dataset))ifi%5==1])
train_ds=Subset(dataset=dataset,indices=[iforiinrange(len(dataset))ifi%5!=1])
查看訓練集
foriinrange(5):
print(train_ds[i])
{'text':'@tiffanylueiknowiwaslistenintobadhabitearlierandistartedfreakinathispart=[','label':0,'qid':'0'}
{'text':'Funeralceremony...gloomyfriday...','label':1,'qid':'2'}
{'text':'wantstohangoutwithfriendsSOON!','label':2,'qid':'3'}
{'text':'@dannycastilloWewanttotradewithsomeonewhohasHoustontickets,butnoonewill.','label':3,'qid':'4'}
{'text':"Ishouldbesleep,butimnot!thinkingaboutanoldfriendwhoIwant.buthe'smarriednow.damn,&hewantsme2!scandalous!",'label':1,'qid':'5'}
在轉換為MapDataset類型
train_ds=MapDataset(train_ds)
dev_ds=MapDataset(dev_ds)
print(len(train_ds))
print(len(dev_ds))
240006000
四、模型選擇
近年來,大量的研究表明基于大型語料庫的預訓練模型(Pretrained Models, PTM)可以學習通用的語言表示,有利于下游NLP任務,同時能夠避免從零開始訓練模型。隨著計算能力的發展,深度模型的出現(即 Transformer)和訓練技巧的增強使得 PTM 不斷發展,由淺變深。
情感預訓練模型SKEP(Sentiment Knowledge Enhanced Pre-training for Sentiment Analysis)。SKEP利用情感知識增強預訓練模型, 在14項中英情感分析典型任務上全面超越SOTA,此工作已經被ACL 2020錄用。SKEP是百度研究團隊提出的基于情感知識增強的情感預訓練算法,此算法采用無監督方法自動挖掘情感知識,然后利用情感知識構建預訓練目標,從而讓機器學會理解情感語義。SKEP為各類情感分析任務提供統一且強大的情感語義表示。
百度研究團隊在三個典型情感分析任務,句子級情感分類(Sentence-level Sentiment Classification),評價目標級情感分類(Aspect-level Sentiment Classification)、觀點抽取(Opinion Role Labeling),共計14個中英文數據上進一步驗證了情感預訓練模型SKEP的效果。
具體實驗效果參考:https://github.com/baidu/Senta#skep
PaddleNLP已經實現了SKEP預訓練模型,可以通過一行代碼實現SKEP加載。
句子級情感分析模型是SKEP fine-tune 文本分類常用模型SkepForSequenceClassification。其首先通過SKEP提取句子語義特征,之后將語義特征進行分類。
!pipinstallregex
Lookinginindexes:https://mirror.baidu.com/pypi/simple/
Requirementalreadysatisfied:regexin/opt/conda/envs/python35-paddle120-env/lib/python3.7/site-packages(2021.8.28)
1.Skep模型加載
SkepForSequenceClassification可用于句子級情感分析和目標級情感分析任務。其通過預訓練模型SKEP獲取輸入文本的表示,之后將文本表示進行分類。
pretrained_model_name_or_path:模型名稱。支持"skep_ernie_1.0_large_ch",“skep_ernie_2.0_large_en”。
** “skep_ernie_1.0_large_ch”:是SKEP模型在預訓練ernie_1.0_large_ch基礎之上在海量中文數據上繼續預訓練得到的中文預訓練模型;
“skep_ernie_2.0_large_en”:是SKEP模型在預訓練ernie_2.0_large_en基礎之上在海量英文數據上繼續預訓練得到的英文預訓練模型;
num_classes: 數據集分類類別數。
關于SKEP模型實現詳細信息參考:https://github.com/PaddlePaddle/PaddleNLP/tree/develop/paddlenlp/transformers/skep
from paddlenlp.transformers import SkepForSequenceClassification, SkepTokenizer
指定模型名稱,一鍵加載模型
model=SkepForSequenceClassification.from_pretrained(pretrained_model_name_or_path="skep_ernie_2.0_large_en",num_classes=13)
同樣地,通過指定模型名稱一鍵加載對應的Tokenizer,用于處理文本數據,如切分token,轉token_id等。
tokenizer=SkepTokenizer.from_pretrained(pretrained_model_name_or_path="skep_ernie_2.0_large_en")
[2021-09-161058,665][INFO]-Alreadycached/home/aistudio/.paddlenlp/models/skep_ernie_2.0_large_en/skep_ernie_2.0_large_en.pdparams
[2021-09-161010,133][INFO]-Found/home/aistudio/.paddlenlp/models/skep_ernie_2.0_large_en/skep_ernie_2.0_large_en.vocab.txt
2.引入可視化VisualDl
fromvisualdlimportLogWriter
writer=LogWriter("./log")
3.數據處理
SKEP模型對文本處理按照字粒度進行處理,我們可以使用PaddleNLP內置的SkepTokenizer完成一鍵式處理。
defconvert_example(example,
tokenizer,
max_seq_length=512,
is_test=False):
#將原數據處理成model可讀入的格式,enocded_inputs是一個dict,包含input_ids、token_type_ids等字段
encoded_inputs=tokenizer(
text=example["text"],max_seq_len=max_seq_length)
# input_ids:對文本切分token后,在詞匯表中對應的token id
input_ids=encoded_inputs["input_ids"]
# token_type_ids:當前token屬于句子1還是句子2,即上述圖中表達的segment ids
token_type_ids=encoded_inputs["token_type_ids"]
ifnotis_test:
# label:情感極性類別
label=np.array([example["label"]],dtype="int64")
returninput_ids,token_type_ids,label
else:
# qid:每條數據的編號
qid=np.array([example["qid"]],dtype="int64")
returninput_ids,token_type_ids,qid
defcreate_dataloader(dataset,
trans_fn=None,
mode='train',
batch_size=1,
batchify_fn=None):
iftrans_fn:
dataset=dataset.map(trans_fn)
shuffle=Trueifmode=='train'elseFalse
ifmode=="train":
sampler=paddle.io.DistributedBatchSampler(
dataset=dataset,batch_size=batch_size,shuffle=shuffle)
else:
sampler=paddle.io.BatchSampler(
dataset=dataset,batch_size=batch_size,shuffle=shuffle)
dataloader=paddle.io.DataLoader(
dataset,batch_sampler=sampler,collate_fn=batchify_fn)
returndataloader
4.評估函數定義
importnumpyasnp
importpaddle
@paddle.no_grad()
defevaluate(model,criterion,metric,data_loader):
model.eval()
metric.reset()
losses=[]
forbatchindata_loader:
input_ids,token_type_ids,labels=batch
logits=model(input_ids,token_type_ids)
loss=criterion(logits,labels)
losses.append(loss.numpy())
correct=metric.compute(logits,labels)
metric.update(correct)
accu=metric.accumulate()
#print("evalloss:%.5f,accu:%.5f"%(np.mean(losses),accu))
model.train()
metric.reset()
returnnp.mean(losses),accu
5.超參定義
定義損失函數、優化器以及評價指標后,即可開始訓練。
推薦超參設置:
batch_size=100
max_seq_length=166
batch_size=100
learning_rate=4e-5
epochs=32
warmup_proportion=0.1
weight_decay=0.01
實際運行時可以根據顯存大小調整batch_size和max_seq_length大小。
importos
fromfunctoolsimportpartial
importnumpyasnp
importpaddle
importpaddle.nn.functionalasF
frompaddlenlp.dataimportStack,Tuple,Pad
#批量數據大小
batch_size=100
#文本序列最大長度166
max_seq_length=166
#批量數據大小
batch_size=100
#定義訓練過程中的最大學習率
learning_rate=4e-5
#訓練輪次
epochs=32
#學習率預熱比例
warmup_proportion=0.1
#權重衰減系數,類似模型正則項策略,避免模型過擬合
weight_decay=0.01
將數據處理成模型可讀入的數據格式
trans_func=partial(
convert_example,
tokenizer=tokenizer,
max_seq_length=max_seq_length)
將數據組成批量式數據,如將不同長度的文本序列padding到批量式數據中最大長度將每條數據label堆疊在一起
batchify_fn=lambdasamples,fn=Tuple(
Pad(axis=0,pad_val=tokenizer.pad_token_id),#input_ids
Pad(axis=0,pad_val=tokenizer.pad_token_type_id),#token_type_ids
Stack()#labels
):[datafordatainfn(samples)]
train_data_loader=create_dataloader(
train_ds,
mode='train',
batch_size=batch_size,
batchify_fn=batchify_fn,
trans_fn=trans_func)
dev_data_loader=create_dataloader(
dev_ds,
mode='dev',
batch_size=batch_size,
batchify_fn=batchify_fn,
trans_fn=trans_func)
定義超參,loss,優化器等
frompaddlenlp.transformersimportLinearDecayWithWarmup
importtime
num_training_steps=len(train_data_loader)*epochs
lr_scheduler=LinearDecayWithWarmup(learning_rate,num_training_steps,warmup_proportion)
AdamW優化器
optimizer=paddle.optimizer.AdamW(
learning_rate=lr_scheduler,
parameters=model.parameters(),
weight_decay=weight_decay,
apply_decay_param_fun=lambdax:xin[
p.nameforn,pinmodel.named_parameters()
ifnotany(ndinnforndin["bias","norm"])
])
criterion=paddle.nn.loss.CrossEntropyLoss()#交叉熵損失函數
metric=paddle.metric.Accuracy()#accuracy評價指標
五、訓練
訓練且保存最佳結果
開啟訓練
global_step=0
best_val_acc=0
tic_train=time.time()
best_accu=0
forepochinrange(1,epochs+1):
forstep,batchinenumerate(train_data_loader,start=1):
input_ids,token_type_ids,labels=batch
#喂數據給model
logits=model(input_ids,token_type_ids)
#計算損失函數值
loss=criterion(logits,labels)
#預測分類概率值
probs=F.softmax(logits,axis=1)
#計算acc
correct=metric.compute(probs,labels)
metric.update(correct)
acc=metric.accumulate()
global_step+=1
ifglobal_step%10==0:
print(
"globalstep%d,epoch:%d,batch:%d,loss:%.5f,accu:%.5f,speed:%.2fstep/s"
%(global_step,epoch,step,loss,acc,
10/(time.time()-tic_train)))
tic_train=time.time()
#反向梯度回傳,更新參數
loss.backward()
optimizer.step()
lr_scheduler.step()
optimizer.clear_grad()
ifglobal_step%100==0and:
#評估當前訓練的模型
eval_loss,eval_accu=evaluate(model,criterion,metric,dev_data_loader)
print("evalondevloss:{:.8},accu:{:.8}".format(eval_loss,eval_accu))
#加入eval日志顯示
writer.add_scalar(tag="eval/loss",step=global_step,value=eval_loss)
writer.add_scalar(tag="eval/acc",step=global_step,value=eval_accu)
#加入train日志顯示
writer.add_scalar(tag="train/loss",step=global_step,value=loss)
writer.add_scalar(tag="train/acc",step=global_step,value=acc)
save_dir="best_checkpoint"
#加入保存
ifeval_accu>best_val_acc:
ifnotos.path.exists(save_dir):
os.mkdir(save_dir)
best_val_acc=eval_accu
print(f"模型保存在{global_step}步,最佳eval準確度為{best_val_acc:.8f}!")
save_param_path=os.path.join(save_dir,'best_model.pdparams')
paddle.save(model.state_dict(),save_param_path)
fh=open('best_checkpoint/best_model.txt','w',encoding='utf-8')
fh.write(f"模型保存在{global_step}步,最佳eval準確度為{best_val_acc:.8f}!")
fh.close()
globalstep10,epoch:1,batch:10,loss:2.64415,accu:0.08400,speed:0.96step/s
globalstep20,epoch:1,batch:20,loss:2.48083,accu:0.09050,speed:0.98step/s
globalstep30,epoch:1,batch:30,loss:2.36845,accu:0.10933,speed:0.98step/s
globalstep40,epoch:1,batch:40,loss:2.24933,accu:0.13750,speed:1.00step/s
globalstep50,epoch:1,batch:50,loss:2.14947,accu:0.15380,speed:0.97step/s
globalstep60,epoch:1,batch:60,loss:2.03459,accu:0.17100,speed:0.96step/s
globalstep70,epoch:1,batch:70,loss:2.23222,accu:0.18414,speed:1.01step/s
visualdl 可視化訓練,時刻掌握訓練走勢,不浪費算力
六、預測
訓練完成后,重啟環境,釋放顯存,開始預測
1.test數據集讀取
數據讀取
importpandasaspd
frompaddlenlp.datasetsimportload_dataset
frompaddle.ioimportDataset,Subset
frompaddlenlp.datasetsimportMapDataset
test=pd.read_csv('/home/mw/input/Twitter4903/test.csv')
數據讀取
defread_test(pd_data):
forindex,iteminpd_data.iterrows():
yield{'text':item['content'],'label':0,'qid':item['tweet_id'].strip('tweet_')}
test_ds=load_dataset(read_test,pd_data=test,lazy=False)
#在轉換為MapDataset類型
test_ds=MapDataset(test_ds)
print(len(test_ds))
defconvert_example(example,
tokenizer,
max_seq_length=512,
is_test=False):
#將原數據處理成model可讀入的格式,enocded_inputs是一個dict,包含input_ids、token_type_ids等字段
encoded_inputs=tokenizer(
text=example["text"],max_seq_len=max_seq_length)
# input_ids:對文本切分token后,在詞匯表中對應的token id
input_ids=encoded_inputs["input_ids"]
# token_type_ids:當前token屬于句子1還是句子2,即上述圖中表達的segment ids
token_type_ids=encoded_inputs["token_type_ids"]
ifnotis_test:
# label:情感極性類別
label=np.array([example["label"]],dtype="int64")
returninput_ids,token_type_ids,label
else:
# qid:每條數據的編號
qid=np.array([example["qid"]],dtype="int64")
returninput_ids,token_type_ids,qid
defcreate_dataloader(dataset,
trans_fn=None,
mode='train',
batch_size=1,
batchify_fn=None):
iftrans_fn:
dataset=dataset.map(trans_fn)
shuffle=Trueifmode=='train'elseFalse
ifmode=="train":
sampler=paddle.io.DistributedBatchSampler(
dataset=dataset,batch_size=batch_size,shuffle=shuffle)
else:
sampler=paddle.io.BatchSampler(
dataset=dataset,batch_size=batch_size,shuffle=shuffle)
dataloader=paddle.io.DataLoader(
dataset,batch_sampler=sampler,collate_fn=batchify_fn)
returndataloader
2.模型加載
frompaddlenlp.transformersimportSkepForSequenceClassification,SkepTokenizer
指定模型名稱,一鍵加載模型
model=SkepForSequenceClassification.from_pretrained(pretrained_model_name_or_path="skep_ernie_2.0_large_en",num_classes=13)
同樣地,通過指定模型名稱一鍵加載對應的Tokenizer,用于處理文本數據,如切分token,轉token_id等。
tokenizer=SkepTokenizer.from_pretrained(pretrained_model_name_or_path="skep_ernie_2.0_large_en")
fromfunctoolsimportpartial
importnumpyasnp
importpaddle
importpaddle.nn.functionalasF
frompaddlenlp.dataimportStack,Tuple,Pad
batch_size=16
max_seq_length=166
#處理測試集數據
trans_func=partial(
convert_example,
tokenizer=tokenizer,
max_seq_length=max_seq_length,
is_test=True)
batchify_fn=lambdasamples,fn=Tuple(
Pad(axis=0,pad_val=tokenizer.pad_token_id),#input
Pad(axis=0,pad_val=tokenizer.pad_token_type_id),#segment
Stack()#qid
):[datafordatainfn(samples)]
test_data_loader=create_dataloader(
test_ds,
mode='test',
batch_size=batch_size,
batchify_fn=batchify_fn,
trans_fn=trans_func)
加載模型
importos
#根據實際運行情況,更換加載的參數路徑
params_path='best_checkpoint/best_model.pdparams'
ifparams_pathandos.path.isfile(params_path):
#加載模型參數
state_dict=paddle.load(params_path)
model.set_dict(state_dict)
print("Loadedparametersfrom%s"%params_path)
3.數據預測
results=[]
#切換model模型為評估模式,關閉dropout等隨機因素
model.eval()
forbatchintest_data_loader:
input_ids,token_type_ids,qids=batch
#喂數據給模型
logits=model(input_ids,token_type_ids)
#預測分類
probs=F.softmax(logits,axis=-1)
idx=paddle.argmax(probs,axis=1).numpy()
idx=idx.tolist()
qids=qids.numpy().tolist()
results.extend(zip(qids,idx))
4.保存并提交
#寫入預測結果,提交
withopen("submission.csv",'w',encoding="utf-8")asf:
#f.write("數據ID,評分
")
f.write("tweet_id,label
")
for(idx,label)inresults:
f.write('tweet_'+str(idx[0])+","+str(label)+"
")
七、注意事項
- 1.使用pandas讀取平面文件相對方便
- 2.max_seq_length用pandas統計最大值出來較為合適
- 3.用pandas可以分析數據分布
- 4.PaddleNLP在自然語言處理方面,有特別多的積累,特別方便,可上github了解
八、PaddleNLP是什么?
1.gitee地址
https://gitee.com/paddlepaddle/PaddleNLP/blob/develop/README.md
2.簡介
PaddleNLP 2.0是飛槳生態的文本領域核心庫,具備易用的文本領域API,多場景的應用示例、和高性能分布式訓練三大特點,旨在提升開發者文本領域的開發效率,并提供基于飛槳2.0核心框架的NLP任務最佳實踐。
基于飛槳核心框架領先的自動混合精度優化策略,結合分布式Fleet API,支持4D混合并行策略,可高效地完成超大規模參數的模型訓練。-
nlp
+關注
關注
1文章
489瀏覽量
22093 -
paddle
+關注
關注
0文章
4瀏覽量
2021
原文標題:八、PaddleNLP是什么?
文章出處:【微信號:zenRRan,微信公眾號:深度學習自然語言處理】歡迎添加關注!文章轉載請注明出處。
發布評論請先 登錄
相關推薦
關于SMT回流焊接,你了解多少?
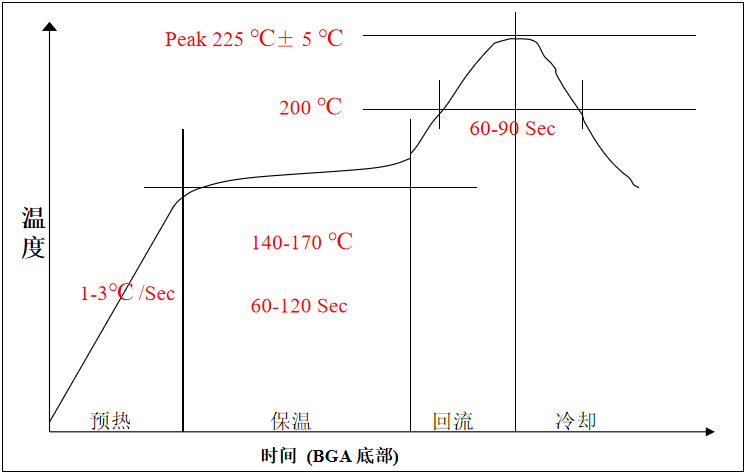
關于SMT回流焊接,你了解多少?
關于磁通計你了解多少?
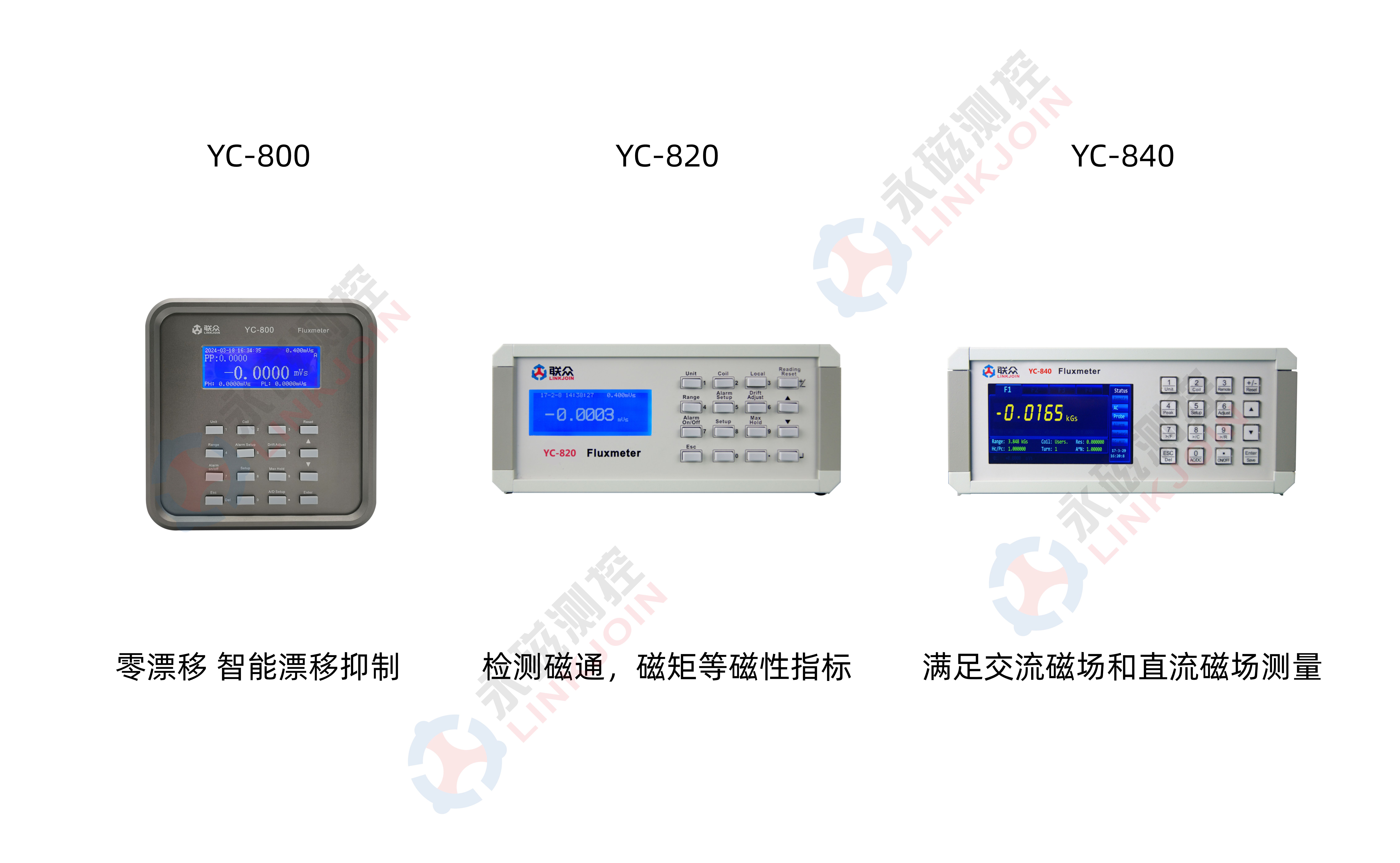
這些關于IP地址定位術語你了解嗎?
關于LCD應用,你都了解什么?
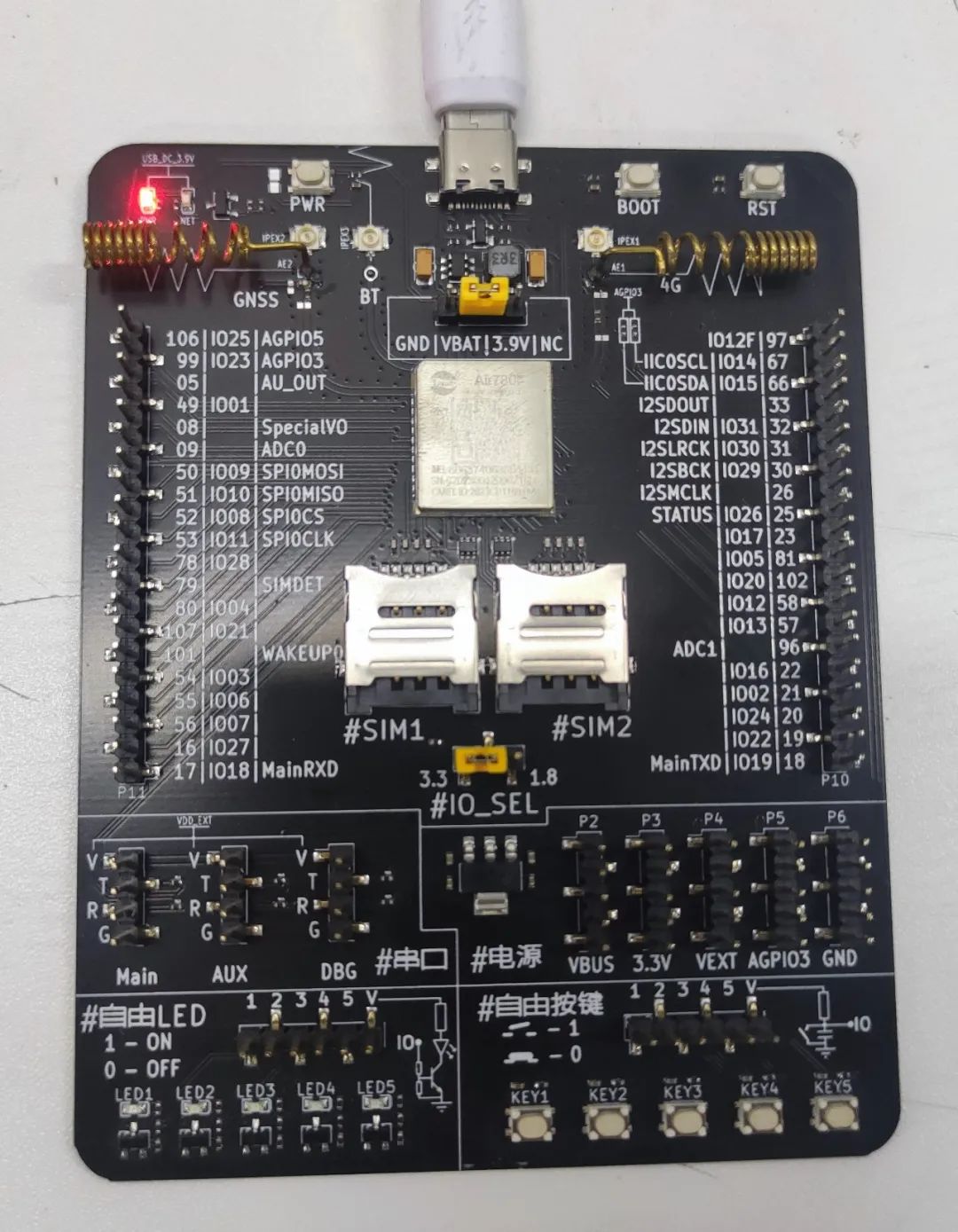
浪潮信息源2.0大模型與百度PaddleNLP全面適配
關于工廠人員定位,這幾點你了解嗎?
關于LT8711UXD你了解多少?
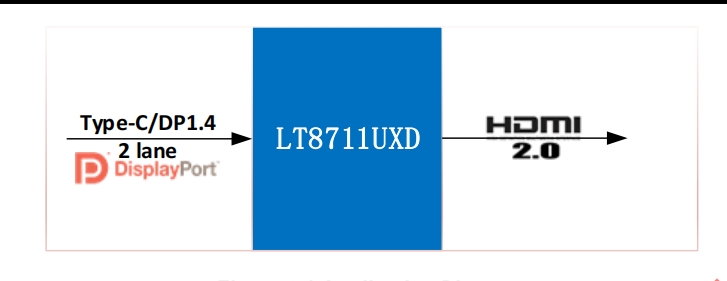
MINIWARE的品牌故事,你了解多少?
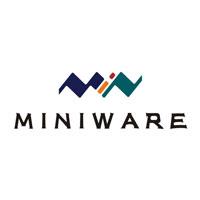
你真的了解駐波比嗎?到底什么是電壓駐波比?
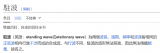
關于ECU 和 MCU ,你了解多少?
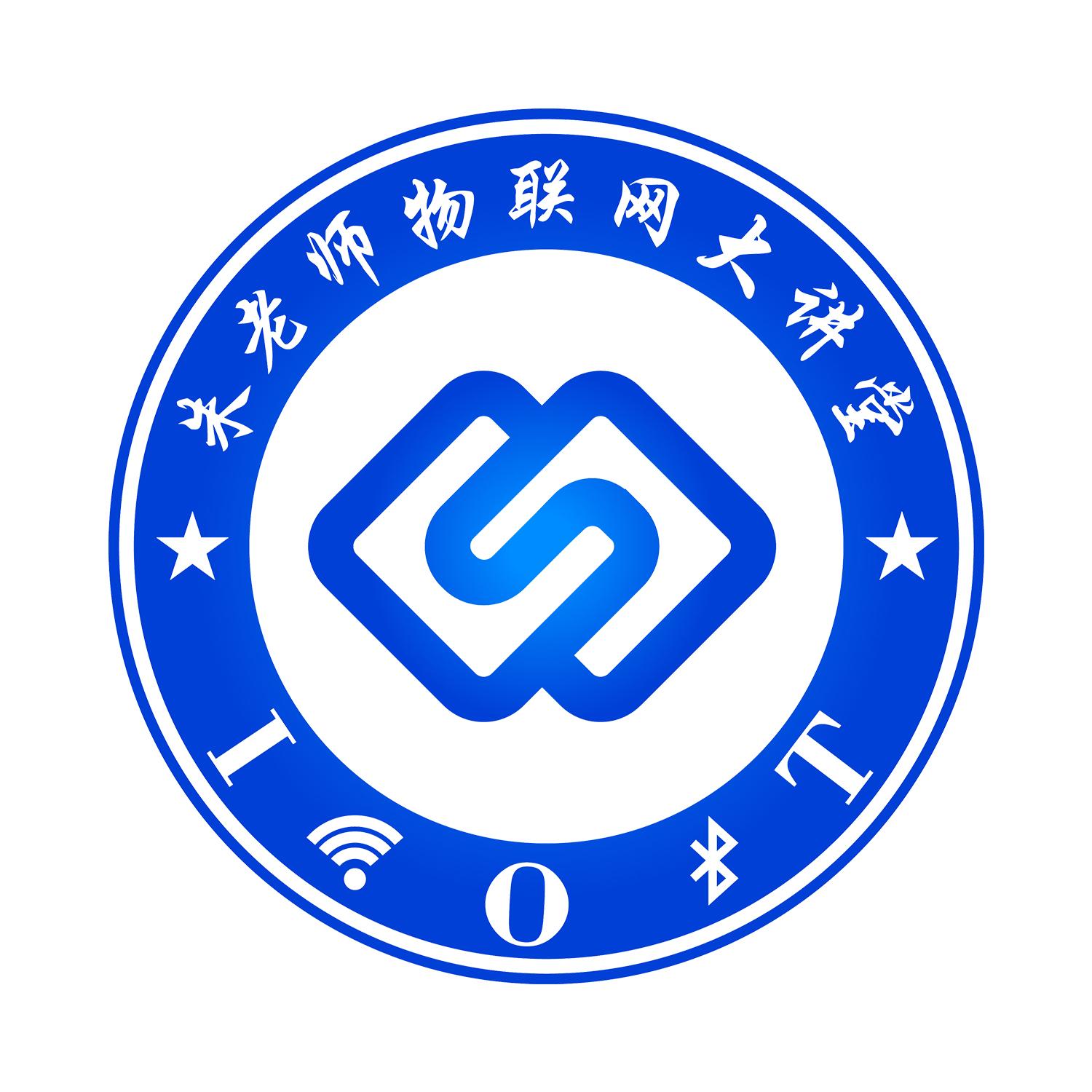
評論